AI and Recidvism
Contents
Introduction
When it comes to criminal justice, the United States incorporates a variety of AI tools to assess criminal risk levels. These machine-learning based algorithms use statistical data to find patterns in criminal behavior [1]. These patterns intend to aid police in prioritizing resources as well as predicting future criminal behavior and criminal risk associated with individuals. Recidivism refers to the tendency of a convicted criminal to commit a future crime post-conviction[2].
Law enforcement uses tools to assess potential recidivism in defendants to influence their decision-making process. It is important for law enforcement to theorize potential risk levels for criminal defendants because incarceration rates remain high, and resources remain low. [3] A criminal deemed less risky by recidivism AI is given more options for routes like community service, parole, and shorter sentencing. On the other hand, a criminal deemed risky by recidivism AI may have fewer options for parole, re-release, or shorter sentencing since it is perceived they will commit future crimes. These future crimes range from anything from violent crime, to property crime, to felony charges, and misdemeanor charges. [4].
Predicting levels of recidivism is important to law enforcement due to a variety of outside factors. These factors include, but are not limited to, pressure to lower incarceration rates in the United States and limited resources for law enforcement. Currently, the United States incarcerates at the sixth highest rate in the world [5]. The sheer volume of offenders that need to be processed through the legal system created the demand for a quick, unbiased software to help law makers decide which criminals are most dangerous or risky. This would allow them to optimize resources. Especially since the US prison population is so high, as mentioned before, the demand for a software to streamline the legal process created room for recidivism software to enter the market. Predicting recidivism is one of the tools law makers use to assess risk and influence their sentencing decision making. As the United States faces political pressure to lower incarceration rates, AI software seeks to prioritize the “riskiest” criminals for incarceration and allocate resources appropriately to reflect criminal risk.
COMPAS
The most popular machine learning algorithm used in this practice is COMPAS, standing for Correctional Offender Management Profiling for Alternative Sanctions (COMPAS) [6].
This particular risk assessment machine learning algorithm tool aims to predict levels of recidivism using a total of 43 scales and assesses 3 types of predicted recidivism [7].
COMPAS was developed by Tim Brennan and David Wells. COMPAS was founded in 1989 [8]. The former was a PhD in Behavioral Science and former Professor at University of Colorado and the latter was a former corrections officer in Traverse City, MI. [9]
COMPAS is a for-profit software under the company Equivant (formerly Northpointe). Equivant is a software company specializing in justice software. [10] It is not intended for public use.
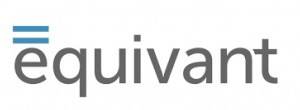
COMPAS entered the market in 1998. [11] It is unknown exactly how many court systems use COMPAS.
The core data for COMPAS was sampled from over “30,000 COMPAS Core assessments conducted between January 2004 and November 2005 at prison, parole, jail and probation sites across the United States.” The tool does not “dynamically learn through new data after implantation”. Instead, the machine learning data the algorithm pulls from must be updated manually. [12]
The stages of intended use are pretrial release, prison, and parole. [13]
Pretrial Release Risk Scale
The pretrial release risk scale (PRRS) is a risk assessment tool done by COMPAS to predict failure to appear in court and felony arrest during pretrial release. [14] Applications of the PRRS include sorting pretrial caseload in to low, moderate, and high risk groups “based on the likelihood of failure to appear in court or commit a new crime pending trial.” [15]. It is currently used in states like California, Wisconsin, and New York. [16]
General Recidivism Risk Scale
The general recidivism scale seeks to assess the likelihood of a misdemeanor or felony 2 years from the date of assessment. [17] “The scale inputs include criminal involvement (prior arrests and prior sentences to jail, prison, and probation), vocational/educational problems, drug history, age-at-assessment, and age-at-first-arrest. All of these risk factors are well known predictors of recidivism.” [18]. The scores are given on a scale of 1 to 10 with 1 to 4 being low risk, 5 to 7 mid level risk, and 8 to 10 high level risk [19].
Violent Recidivism Risk Scale
The violent recidivism risk scale seeks to assess the likelihood of a violent crime within 2 years from the date of assessment [20]. The scale inputs are very similar to the general recidivism scale but also considers history of violence and noncompliance. [21] The scores are given on a scale of 1 to 10 with 1 to 4 being low risk, 5 to 7 mid level risk, and 8 to 10 high level risk [22]. Individuals deemed high risk may be placed under “special supervision conditions.” [23] The violent recidivism scale is very influential in the decision making process since perceived potentially violent defenders require more resources and attention by law enforcement, as reflected by the "special supervision conditions" stated above.
Factors
COMPAS uses a variety of factors to generate their risk score [24]. Some of these factors include but are not limited to family criminal history, financial situation, financial history, financial literacy, history of violence and non-compliance, social environment, substance abuse, education and vocation, length of residency, location of residency, leisure time and affinity for boredom, personality, criminal opportunity, social network(friends and family criminal records), and many others [25]. It is important to note that some of these factors, such as personality and affinity for boredom, are subjective and not rooted in fact.
Usage
Many state and local governments use or have used COMPAS including Wisconsin, Florida, New York, and California. [26] The Wisconsin government website has a section on COMPAS reading “Evidence Based Practices clearly state that having a sound assessment that accurately identifies an offender’s risk to reoffend is the cornerstone of effective supervision. Without a proper assessment, appropriate interventions and services cannot be delivered. Services are targeted for moderate to higher-risk offenders who are likely to reoffend if appropriate interventions are not available.” [27] It is important to note that governments like Wisconsin and California are in favor of this software. Since COMPAS allows them to sort individuals by perceived riskiness, they can allocate time and resources more effectively [28]. This one of the reasons the demand for a software like COMPAS is so high since overwhelmed justice systems can use it to officially allocate its resources. Wisconsin’s statement on their website reflects law enforcement and legal enforcement’s desire for a tool like COMPAS to streamline their decision making processes.
Training
COMPAS requires sixteen total hours of training. The training takes place over the course of two days. Training is required by any government or court system who wishes to use the COMPAS software. [29]
State v. Loomis
In 2013, Eric Loomis was charged with 5 criminal counts in relation to a drive by shooting in La Crosse. [30] Loomis denied participating in the shooting and plead guilty to two lighter charges. [31] The state of Wisconsin used COMPAS to asses Loomis’ risk. Loomis received high risk scores in all three categories. [32] The COMPAS score influenced the courts to sentence Loomis to six years in prison and five years under supervision. [33] Loomis then filed a motion arguing that “the court’s reliance on COMPAS violated his due process rights… COMPAS assessment infringed on both his right to an individualized sentence and his right to be sentenced on accurate information Loomis additionally argued on due process grounds that the court unconstitutionally considered gender at sentencing by relying on a risk assessment that took gender into account. The trial court denied the post-conviction motion, and the Wisconsin Court of Appeals certified the appeal to the Wisconsin Supreme Court.”[34]
Ultimately, the Wisconsin Supreme Court reject Loomis’ argument. [35] Justice Bradley stated “the use of gender as a factor in the risk assessment served the nondiscriminatory purpose of promoting accuracy and that Loomis had not provided sufficient evidence that the sentencing court had actually considered gender. Moreover, as COMPAS uses only publicly available data and data provided by the defendant, the court concluded that Loomis could have denied or explained any information that went into making the report and therefore could have verified the accuracy of the information used in sentencing.” [36] This case was significant since it brought to light that COMPAS relies on group data and not individual data which raised ethical concerns.
Other AI Recidivism Tools
Many states in the United States have their own unique tools for assessing recidivism risk. [37]. For example, California uses California Static Risk Assessment (CSRA). [38] In their frequently asked questions page on the CSRA, the California government states, “The California Static Risk Assessment (CSRA) uses an incarcerated individuals past criminal history and characteristics to predict their risk to reoffend.”[39] However, the California government uses COMPAS as an essential part of the CSRA, stating, “IMPORTANT: The COMPAS assessment is one of the most influential tools CDCR uses to determine an incarcerated individuals rehabilitative needs and will be administered repeatedly throughout the incarcerated individuals stay in prison.” [40] While other recidivism tools exist, COMPAS remains the most influential of them all. California is a strong example of this. California’s statement on COMPAS, similar to Wisconsin’s, reflects the high demand for a software that can predict recidivism levels amongst defendants.
Concerns Over Bias
COMPAS was designed with the intent to be free of bias [41]. The machine learning algorithm takes a breadth of data and asses a variety of factors based on this data. The algorithm was built to be free of human bias. Since COMPAS looked at data, it was perceived to be fair and free of human biases [42].
Additionally, software like COMPAS aim to eliminate human bias associated with predicting recidivism. The logic behind these AI software goes something like this: humans can possess unconscious bias that may unfairly influence how judges predict recidivism. A machine learning AI, on the other hand, can only assess the data.
While the theory behind recidivism predicting AI software is aimed to eliminate bias, the implementation of COMPAS has many limitations that disproportionately hurt certain groups of people[43]. For example, the recidivism AI COMPAS assesses a defendant’s risk of committing either a misdemeanor or felony in the next 2 years from assessment [44]. COMPAS analyzes everything from housing stability, substance use, family income levels, community ties, employment status, and more. Lower levels of factors like housing stability, family income levels, and employment status and income often correlate to race. Because of this correlation, the recidivism AI inaccurately predicted higher recidivism rates among black defendants. “Black defendants who did not recidivate were incorrectly predicted to reoffend at a rate of 44.9%, nearly twice as high as their white counterparts at 23.5%” [45] Additionally, in the case of State v. Loomis, Loomis raised concerns over using gender as a basis for assessing recidivism risk[46].
This insight in to COMPAS exposes the limitations present in recidivism AI. While the software sought to discourage racial bias in criminal sentencing, it furthered the gap in inequitable sentencing particularly between black and white defendants. Software like COMPAS cannot distinguish between correlation and causation. Family income, employment levels, and other factors COMPAS analyzes unfortunately fall under the umbrella of correlating to generations of systemic racism. Because of this, the rates of unemployment are higher amongst black populations than white in the United States amongst many other trends that COMPAS analyzes. While unemployment may correlate to certain types of crimes, such as property crimes, using factors such as this unfairly and inaccurately predict higher rates of recidivism amongst black defendants. This and many other factors can explain the discrepancy in black and white recidivism prediction accuracies.
Furthermore, the data available for machine learning algorithms such as COMPAS to analyze is skewed. Years of sentencing inequity disproportionately and unjustly deemed black offenders riskier, ranging from predicting higher likelihood for crimes as well as higher likelihood for violent crimes. The data COMPAS learns from reflects this inequity. In short, the historical data COMPAS learns patterns from is biased, making the algorithm biased as well. Learning from biased data will encourage software like COMPAS to make learn from these biased patterns and reinforce them. Additionally, since new data must be entered manually which happens infrequently, [47] it can be inferred that the data COMPAS learns from may be outdated and reflect outdated trends or beliefs against certain groups. Furthermore, the data was collected by the two creators. [48] If the two creators had any unconscious biases, that would be reflected in the data they chose to include in COMPAS’ machine learning. This could lead to statistical bias. For example, if COMPAS had a set of data to learn from and that set of data was skewed to include more data on certain groups of people, then COMPAS would reinforce those patterns. Additionally, only 30,000 samples were collected [49] when the estimated priosn population remains over 2 million [50]. This is only 1.5% of the prison population. The sample may include sampling biases due to the small size of their sample population. Additionally, as noted previously in this article, the data is not automatically updated. So any biases persistent in the original collected data set will likely remain. It would be beneficial to increase the sample size as well as continuing to update COMPAS' learning data set.
Depending on how and what data was collected, this would impact COMPAS’ machine learning outcomes.
Conclusion
COMPAS discourages fair sentencing because it cannot distinguish truth from correlation. Since COMPAS looks at trends amongst groups, it enforces certain stereotypes across race and gender. For example, considering factors like length of residency and property value present biases against those living below the poverty line. This can also be correlated to race and ethnicity, which is why certain groups are disproportionately negatively affected. Systemic racism has created a cycle of poverty amongst minority groups unrelated to violence and crime. Yet, COMPAS uses these factors as indicators of risk. These stereotypes then influence judge decision making and can have serious unintended negative consequences. When assessing the data available to it, COMPAS unjustly and inaccurately perceives black offenders as riskier than white offenders. This perpetuates existing racial inequities in prison sentencing and allows sentencing inequity to thrive.
Ultimately, there is a lot of controversy surrounding the use of tools like COMPAS. However, the overwhelming demand for such a software keeps this software remaining in practice.
References
- ↑ (Hao, K. (2020, April 2). Ai is sending people to jail-and getting it wrong. MIT Technology Review. Retrieved January 27, 2023, from https://www.technologyreview.com/2019/01/21/137783/algorithms-criminal-justice-ai/ )
- ↑ policy)., N. I. J. (see reuse. (n.d.). Recidivism. National Institute of Justice. Retrieved February 10, 2023, from https://nij.ojp.gov/topics/corrections/recidivism
- ↑ (Published by Statista Research Department, & 3, J. (2023, January 3). Ranking: Most prisoners per capita by country 2023. Statista. Retrieved January 27, 2023, from https://www.statista.com/statistics/262962/countries-with-the-most-prisoners-per-100-000-inhabitants/ )
- ↑ Practitioner’s Guide to Compas Core - Equivant. (n.d.). Retrieved February 10, 2023, from https://www.equivant.com/wp-content/uploads/Practitioners-Guide-to-COMPAS-Core-040419.pdf
- ↑ (Published by Statista Research Department, & 3, J. (2023, January 3). Ranking: Most prisoners per capita by country 2023. Statista. Retrieved January 27, 2023, from https://www.statista.com/statistics/262962/countries-with-the-most-prisoners-per-100-000-inhabitants/ )
- ↑ (Larson, J., Angwin, J., Kirchner, L., & Mattu, S. (2016, May 23). How we analyzed the compass recidivism algorithm. ProPublica. Retrieved January 27, 2023, from https://www.propublica.org/article/how-we-analyzed-the-compas-recidivism-algorithm )
- ↑ Practitioner’s Guide to Compas Core - Equivant. (n.d.). Retrieved February 10, 2023, from https://www.equivant.com/wp-content/uploads/Practitioners-Guide-to-COMPAS-Core-040419.pdf
- ↑ Angwin, J., Larson, J., Kirchner, L., & Mattu, S. (2016, May 23). Machine bias. ProPublica. Retrieved February 10, 2023, from https://www.propublica.org/article/machine-bias-risk-assessments-in-criminal-sentencing
- ↑ Risk assessment tool database. (n.d.). Retrieved February 10, 2023, from https://criminaljustice.tooltrack.org/tool/16627
- ↑ Risk assessment tool database. (n.d.). Retrieved February 10, 2023, from https://criminaljustice.tooltrack.org/tool/16627
- ↑ Risk assessment tool database. (n.d.). Retrieved February 10, 2023, from https://criminaljustice.tooltrack.org/tool/16627
- ↑ Risk assessment tool database. (n.d.). Retrieved February 10, 2023, from https://criminaljustice.tooltrack.org/tool/16627
- ↑ Risk assessment tool database. (n.d.). Retrieved February 10, 2023, from https://criminaljustice.tooltrack.org/tool/16627
- ↑ Practitioner’s Guide to Compas Core - Equivant. (n.d.). Retrieved February 10, 2023, from https://www.equivant.com/wp-content/uploads/Practitioners-Guide-to-COMPAS-Core-040419.pdf
- ↑ Practitioner’s Guide to Compas Core - Equivant. (n.d.). Retrieved February 10, 2023, from https://www.equivant.com/wp-content/uploads/Practitioners-Guide-to-COMPAS-Core-040419.pdf
- ↑ Risk assessment tool database. (n.d.). Retrieved February 10, 2023, from https://criminaljustice.tooltrack.org/tool/16627
- ↑ Practitioner’s Guide to Compas Core - Equivant. (n.d.). Retrieved February 10, 2023, from https://www.equivant.com/wp-content/uploads/Practitioners-Guide-to-COMPAS-Core-040419.pdf
- ↑ Practitioner’s Guide to Compas Core - Equivant. (n.d.). Retrieved February 10, 2023, from https://www.equivant.com/wp-content/uploads/Practitioners-Guide-to-COMPAS-Core-040419.pdf
- ↑ Practitioner’s Guide to Compas Core - Equivant. (n.d.). Retrieved February 10, 2023, from https://www.equivant.com/wp-content/uploads/Practitioners-Guide-to-COMPAS-Core-040419.pdf
- ↑ Practitioner’s Guide to Compas Core - Equivant. (n.d.). Retrieved February 10, 2023, from https://www.equivant.com/wp-content/uploads/Practitioners-Guide-to-COMPAS-Core-040419.pdf
- ↑ Practitioner’s Guide to Compas Core - Equivant. (n.d.). Retrieved February 10, 2023, from https://www.equivant.com/wp-content/uploads/Practitioners-Guide-to-COMPAS-Core-040419.pdf
- ↑ Practitioner’s Guide to Compas Core - Equivant. (n.d.). Retrieved February 10, 2023, from https://www.equivant.com/wp-content/uploads/Practitioners-Guide-to-COMPAS-Core-040419.pdf
- ↑ Practitioner’s Guide to Compas Core - Equivant. (n.d.). Retrieved February 10, 2023, from https://www.equivant.com/wp-content/uploads/Practitioners-Guide-to-COMPAS-Core-040419.pdf
- ↑ Practitioner’s Guide to Compas Core - Equivant. (n.d.). Retrieved February 10, 2023, from https://www.equivant.com/wp-content/uploads/Practitioners-Guide-to-COMPAS-Core-040419.pdf
- ↑ Practitioner’s Guide to Compas Core - Equivant. (n.d.). Retrieved February 10, 2023, from https://www.equivant.com/wp-content/uploads/Practitioners-Guide-to-COMPAS-Core-040419.pdf
- ↑ Compas (software). Wikipedia. Retrieved February 10, 2023, from https://en.wikipedia.org/wiki/COMPAS_(software)
- ↑ Compas. DOC COMPAS. (n.d.). Retrieved February 10, 2023, from https://doc.wi.gov/Pages/AboutDOC/COMPAS.aspx
- ↑ Practitioner’s Guide to Compas Core - Equivant. (n.d.). Retrieved February 10, 2023, from https://www.equivant.com/wp-content/uploads/Practitioners-Guide-to-COMPAS-Core-040419.pdf
- ↑ Risk assessment tool database. (n.d.). Retrieved February 10, 2023, from https://criminaljustice.tooltrack.org/tool/16627
- ↑ State v. Loomis. Harvard Law Review. (2017, March 10). Retrieved February 10, 2023, from https://harvardlawreview.org/2017/03/state-v-loomis/
- ↑ State v. Loomis. Harvard Law Review. (2017, March 10). Retrieved February 10, 2023, from https://harvardlawreview.org/2017/03/state-v-loomis/
- ↑ State v. Loomis. Harvard Law Review. (2017, March 10). Retrieved February 10, 2023, from https://harvardlawreview.org/2017/03/state-v-loomis/
- ↑ State v. Loomis. Harvard Law Review. (2017, March 10). Retrieved February 10, 2023, from https://harvardlawreview.org/2017/03/state-v-loomis/
- ↑ State v. Loomis. Harvard Law Review. (2017, March 10). Retrieved February 10, 2023, from https://harvardlawreview.org/2017/03/state-v-loomis/
- ↑ State v. Loomis. Harvard Law Review. (2017, March 10). Retrieved February 10, 2023, from https://harvardlawreview.org/2017/03/state-v-loomis/
- ↑ State v. Loomis. Harvard Law Review. (2017, March 10). Retrieved February 10, 2023, from https://harvardlawreview.org/2017/03/state-v-loomis/
- ↑ Frequently asked questions (FAQ). Division of Rehabilitative Programs (DRP). (2022, May 19). Retrieved February 10, 2023, from https://www.cdcr.ca.gov/rehabilitation/faq/
- ↑ Frequently asked questions (FAQ). Division of Rehabilitative Programs (DRP). (2022, May 19). Retrieved February 10, 2023, from https://www.cdcr.ca.gov/rehabilitation/faq/
- ↑ Frequently asked questions (FAQ). Division of Rehabilitative Programs (DRP). (2022, May 19). Retrieved February 10, 2023, from https://www.cdcr.ca.gov/rehabilitation/faq/
- ↑ Frequently asked questions (FAQ). Division of Rehabilitative Programs (DRP). (2022, May 19). Retrieved February 10, 2023, from https://www.cdcr.ca.gov/rehabilitation/faq/
- ↑ (Larson, J., Angwin, J., Kirchner, L., & Mattu, S. (2016, May 23). How we analyzed the compas recidivism algorithm. ProPublica. Retrieved January 27, 2023, from https://www.propublica.org/article/how-we-analyzed-the-compas-recidivism-algorithm )
- ↑ (Larson, J., Angwin, J., Kirchner, L., & Mattu, S. (2016, May 23). How we analyzed the compas recidivism algorithm. ProPublica. Retrieved January 27, 2023, from https://www.propublica.org/article/how-we-analyzed-the-compas-recidivism-algorithm )
- ↑ (Larson, J., Angwin, J., Kirchner, L., & Mattu, S. (2016, May 23). How we analyzed the compas recidivism algorithm. ProPublica. Retrieved January 27, 2023, from https://www.propublica.org/article/how-we-analyzed-the-compas-recidivism-algorithm )
- ↑ (Dressel, J. (n.d.). The accuracy, fairness, and limits of predicting recidivism | science ... Retrieved January 27, 2023, from https://www.science.org/doi/10.1126/sciadv.aao5580 )
- ↑ (Dressel, J. (n.d.). The accuracy, fairness, and limits of predicting recidivism | science ... Retrieved January 27, 2023, from https://www.science.org/doi/10.1126/sciadv.aao5580)
- ↑ State v. Loomis. Harvard Law Review. (2017, March 10). Retrieved February 10, 2023, from https://harvardlawreview.org/2017/03/state-v-loomis/
- ↑ Risk assessment tool database. (n.d.). Retrieved February 10, 2023, from https://criminaljustice.tooltrack.org/tool/16627
- ↑ Risk assessment tool database. (n.d.). Retrieved February 10, 2023, from https://criminaljustice.tooltrack.org/tool/16627
- ↑ Risk assessment tool database. (n.d.). Retrieved February 10, 2023, from https://criminaljustice.tooltrack.org/tool/16627
- ↑ Nazgol Ghandnoosh, P. D. and A. N. (2022, October 18). Research - get the facts. The Sentencing Project. Retrieved February 10, 2023, from https://www.sentencingproject.org/research/