Difference between revisions of "Generative Media"
(→Deepfakes) |
(→Deepfakes: Added in the "how to spot deep fakes sections") |
||
Line 8: | Line 8: | ||
==Deepfakes== | ==Deepfakes== | ||
[[File:DeepFake2021.jpg|thumb|right|"DeepFake videos made with a single image in 2021". <ref>https://www.wired.com/story/deepfakes-getting-better-theyre-easy-spot/ </ref>]] | [[File:DeepFake2021.jpg|thumb|right|"DeepFake videos made with a single image in 2021". <ref>https://www.wired.com/story/deepfakes-getting-better-theyre-easy-spot/ </ref>]] | ||
− | The birth of deepfakes began in late 2017 with the use of artificial intelligence algorithms to insert the faces of famous actresses into adult videos.<ref>L. Whittaker, T. C. Kietzmann, J. Kietzmann and A. Dabirian, "“All Around Me Are Synthetic Faces”: The Mad World of AI-Generated Media," in IT Professional, vol. 22, no. 5, pp. 90-99, 1 Sept.-Oct. 2020, doi: 10.1109/MITP.2020.2985492.</ref> When the deepfake's source code became publicly available, demonstrations of its capabilities were generated and the world sat confused when an impersonation of the 44th president, Barack Obama, seemed to portray him out of character. [https://en.wikipedia.org/wiki/Jordan_Peele Jordan Peele], the comedian behind the demonstration quickly revealed himself and explained the danger of such technology when in the hands of questionable individuals. Today, deepfake algorithms are making strides in progression, in that these systems now only need one picture, to generate fabricated media as | + | The birth of deepfakes began in late 2017 with the use of artificial intelligence algorithms to insert the faces of famous actresses into adult videos.<ref>L. Whittaker, T. C. Kietzmann, J. Kietzmann and A. Dabirian, "“All Around Me Are Synthetic Faces”: The Mad World of AI-Generated Media," in IT Professional, vol. 22, no. 5, pp. 90-99, 1 Sept.-Oct. 2020, doi: 10.1109/MITP.2020.2985492.</ref> When the deepfake's source code became publicly available, demonstrations of its capabilities were generated and the world sat confused when an impersonation of the 44th president, Barack Obama, seemed to portray him out of character. [https://en.wikipedia.org/wiki/Jordan_Peele Jordan Peele], the comedian behind the demonstration quickly revealed himself and explained the danger of such technology when in the hands of questionable individuals. Today, deepfake algorithms are making strides in progression, in that these systems now only need one picture, to generate fabricated media as opposed to a couple of years ago when it took hundreds of pictures for the system to generate videos. <ref>Author: Dane Mitrev (March 2021). ''Few-Shot Adversarial Learning of Realistic Neural Talking Head Models''. Retrieved from https://arxiv.org/pdf/1905.08233.pdf</ref> |
+ | |||
+ | ===How to spot Deepfakes=== | ||
+ | Researchers can identify poor-quality deep fakes quickly due to their poor skin texture mapping, unaligned or inaccurate audio alignment, flickering transposed faces, and hair movement, which is particularly difficult for computers to render. However, there are ways to use computers to examine video content and spot deep fakes. <ref>https://us.norton.com/internetsecurity-emerging-threats-how-to-spot-deepfakes.html</ref> | ||
+ | |||
+ | ====Blinking and Unnatural Eye Movements==== | ||
+ | In a 2018 study aiming to expose AI-generated fake faces by analyzing the eye blinking patterns, researchers Yuezun Li, Ming-Ching Chang, Siwei Lyu created a method to detect and identify abnormal blinking patterns.<ref> Li, Yuezun, Ming-Ching Chang, and Siwei Lyu. "In ictu oculi: Exposing ai generated fake face videos by detecting eye blinking." arXiv preprint arXiv:1806.02877 (2018).</ref> However, while their results were promising, anonymous developers introduced new higher quality Deepfake technology for improving their blinking to pass detection. | ||
+ | |||
+ | ====Emotion==== | ||
+ | A 2020 publication discusses a method where Mittal et al. developed a deep learning network that used emotion to identify deep fakes in video content. When tested against two large deep fake identification testing datasets, they scored 84.4% accuracy on the DFDC dataset and a 96.6% on the DF-TIMT datasets.<ref>Mittal, Trisha, et al. "Emotions Don't Lie: A Deepfake Detection Method using Audio-Visual Affective Cues." arXiv preprint arXiv:2003.06711 (2020).</ref> | ||
+ | The method uses video and audio data from real and fake videos to establish a baseline for emotional responses and phrases. | ||
+ | |||
+ | ====Future==== | ||
+ | The race against deep fakes has become an issue with massive implications with Facebook, apple, google, the FBI, and world governments looking for a solution. Many have made the production of deep fake content an offense in policy and lining the fraudulent behavior's edges.<ref>https://www.theguardian.com/technology/2020/jan/07/facebook-bans-deepfake-videos-in-run-up-to-us-election</ref> Algorithms are used to both create deep fakes and detect them. As the technology to combat deep fakes develops, so will the ability to pass detection. | ||
=="The New Rembrandt"== | =="The New Rembrandt"== |
Revision as of 14:45, 19 March 2021
Generative Media is a process of algorithmically and autonomously generating forms of media, such as art, photographs, music, literature, and any other means of communicating in a creative way. It is media constructed with complex systems of mathematical formulas, which mimic human ingenuity. Generative media goes by many names such as "synthetic media" or "organic media" because it is content created without human intervention. The creation of creative material usually takes a significant amount of time, which generative media streamlines... drastically cutting the creation process time down. The term “synthetic media,” denotes artificially generated or manipulated media, such as synthesized audio, virtual reality, and even advanced digital-image creation, which today is highly believable and “true to life.” Deepfakes, a subset of generative media, is of the most concern today.[1]
Generative art a subset of generative media, is when art is generated algorithmically and autonomously, made possible by the advancements in machine learning and neural networks. Machine learning and neural networks are sophisticated systems that learn to make decisions via a repetitive process called "training." Anyone can then train these algorithmic systems regarding a wide range of contexts. For example, with such a system, an artist would feed it data, such as Leonardo DaVinci's entire painting catalog, while fine tuning the neural net to make the decisions that the artist deems satisfactory, then generate a painting with the specifications that were taught. Individuals practicing this type of art call themselves algorists and many of them argue that generative art is not new and did not stem from the digital age, but was born sometime around the eighth century in the islamic world when they first implemented complex geometric patterns. Algorists also argue that Italians in the Renaissance period implemented a mathematical perspective to generate their paintings.
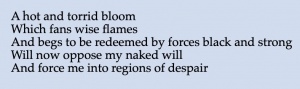
Generative Poetry, a subset of generative media is when algorithms attempt to mimic the meaning, phrasing, structure and rhyme aspects of poetry. Generating algorithmic poetry has been successful in many instances, as Zackary Scholl, from Duke University has demonstrated. He successfully generated poetry that, when posted, received positive feedback. Other engineers have developed systems that use case-based-reasoning to generate formulations of a given input text via a composition of poetic fragments that are retrieved from a case-base of existing poems. The fragments are then configured according to metrical rules, which govern well-formed poetic pieces. Racter is one such program used to generate this synthetic poetry.
Contents
Deepfakes
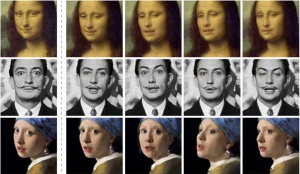
The birth of deepfakes began in late 2017 with the use of artificial intelligence algorithms to insert the faces of famous actresses into adult videos.[4] When the deepfake's source code became publicly available, demonstrations of its capabilities were generated and the world sat confused when an impersonation of the 44th president, Barack Obama, seemed to portray him out of character. Jordan Peele, the comedian behind the demonstration quickly revealed himself and explained the danger of such technology when in the hands of questionable individuals. Today, deepfake algorithms are making strides in progression, in that these systems now only need one picture, to generate fabricated media as opposed to a couple of years ago when it took hundreds of pictures for the system to generate videos. [5]
How to spot Deepfakes
Researchers can identify poor-quality deep fakes quickly due to their poor skin texture mapping, unaligned or inaccurate audio alignment, flickering transposed faces, and hair movement, which is particularly difficult for computers to render. However, there are ways to use computers to examine video content and spot deep fakes. [6]
Blinking and Unnatural Eye Movements
In a 2018 study aiming to expose AI-generated fake faces by analyzing the eye blinking patterns, researchers Yuezun Li, Ming-Ching Chang, Siwei Lyu created a method to detect and identify abnormal blinking patterns.[7] However, while their results were promising, anonymous developers introduced new higher quality Deepfake technology for improving their blinking to pass detection.
Emotion
A 2020 publication discusses a method where Mittal et al. developed a deep learning network that used emotion to identify deep fakes in video content. When tested against two large deep fake identification testing datasets, they scored 84.4% accuracy on the DFDC dataset and a 96.6% on the DF-TIMT datasets.[8] The method uses video and audio data from real and fake videos to establish a baseline for emotional responses and phrases.
Future
The race against deep fakes has become an issue with massive implications with Facebook, apple, google, the FBI, and world governments looking for a solution. Many have made the production of deep fake content an offense in policy and lining the fraudulent behavior's edges.[9] Algorithms are used to both create deep fakes and detect them. As the technology to combat deep fakes develops, so will the ability to pass detection.
"The New Rembrandt"
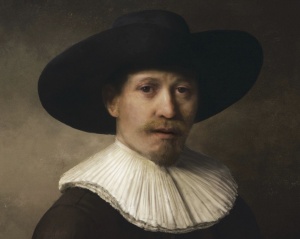
The partition that once existed between fabrication and originality is nearly non existent when analyzing contemporary pieces such as “the Next Rembrandt”. In 2016, a Rembrandt painting was designed by a computer and created by a 3D printer, 351 years after the painter’s death. [11] Tech giant Microsoft and ING Group, a Dutch bank were the two companies that put their abundance of resources together to undertake this endeavor.
Paintings are perceived by many as two dimensional, however, upon thorough analysis of the world's greatest paintings it is revealed that many layers of paint create the third dimension of height. The state-of-the-art algorithms that produced the new Rembrandt created a data set of topological maps that represented brushstrokes and layers of paint, in which the artist used to make the very lifelike depths and tones. [12]
Ethical Dilemmas
Manipulation and credit/copyright issues raise some inevitable ethical dilemmas. There is a melting pot of ideas, conspiracies, prophesies, and feelings that humans have towards artificial intelligence and its capabilities, which makes analyzing its ethical implications essential.
A number of studies have been carried out and are currently taking place to help understand how humans perceive generative media and their feelings towards algorithms in the world of fine arts and literature. "Investigating American and Chinese Subjects’ explicit and implicit perceptions of AI-Generated artistic work" is an academic paper that analyzes one such study, between American and Chinese citizens. [13] The paper reveals a general opinion of artificial intelligence from the public of two of the biggest leaders of technology in the world to get a notion of how much fluctuation there is from one society to the next. The study reports that in China the risks and dangers of fully autonomous systems have not been thoroughly articulated and debated, therefore the majority of Chinese citizens welcome and celebrate the technology, in sharp contrast to Western nations that are cautious with research and development. Public opinion is heavily influenced by the government in China, which makes generative media an efficient outlet to mislead and misinform. The dangerous capabilities of manipulated media will be over looked in societies that show a blind trust to such a novel technology.
Christie's New York, is a prestigious auction house and in 2018 a historical event unfolded. The first ever original work of art generated by artificial intelligence was sold at the final price of $432,500, second to Andy Warhol’s suite of 10 screenprints, surpassing its opening price of $10,000. To successfully generate this piece, algorithms had to go under excessive training by being fed a slew of fine Renaissance paintings from the WikiArt data base, which collected the specifications of its creators, Obvious a Paris based collective, to learn to paint like a master. However, Robbie Barrat, the artist and programmer who wrote the code to generate Renaissance-style images was not amongst those who were compensated for the sale. [14] The relationships that are formed when generating a synthetic piece of art get very complex, which obscures the proportions of involvement, subsequently splitting the reward in an unfair and unethical fashion.
Anthropomorphized algorithmic systems is another ethical issue that arises.
References
- ↑ L. Whittaker, T. C. Kietzmann, J. Kietzmann and A. Dabirian, "“All Around Me Are Synthetic Faces”: The Mad World of AI-Generated Media," in IT Professional, vol. 22, no. 5, pp. 90-99, 1 Sept.-Oct. 2020, doi: 10.1109/MITP.2020.2985492.
- ↑ http://www.101bananas.com/poems/racter.html.
- ↑ https://www.wired.com/story/deepfakes-getting-better-theyre-easy-spot/
- ↑ L. Whittaker, T. C. Kietzmann, J. Kietzmann and A. Dabirian, "“All Around Me Are Synthetic Faces”: The Mad World of AI-Generated Media," in IT Professional, vol. 22, no. 5, pp. 90-99, 1 Sept.-Oct. 2020, doi: 10.1109/MITP.2020.2985492.
- ↑ Author: Dane Mitrev (March 2021). Few-Shot Adversarial Learning of Realistic Neural Talking Head Models. Retrieved from https://arxiv.org/pdf/1905.08233.pdf
- ↑ https://us.norton.com/internetsecurity-emerging-threats-how-to-spot-deepfakes.html
- ↑ Li, Yuezun, Ming-Ching Chang, and Siwei Lyu. "In ictu oculi: Exposing ai generated fake face videos by detecting eye blinking." arXiv preprint arXiv:1806.02877 (2018).
- ↑ Mittal, Trisha, et al. "Emotions Don't Lie: A Deepfake Detection Method using Audio-Visual Affective Cues." arXiv preprint arXiv:2003.06711 (2020).
- ↑ https://www.theguardian.com/technology/2020/jan/07/facebook-bans-deepfake-videos-in-run-up-to-us-election
- ↑ https://www.nextrembrandt.com.
- ↑ United Nations Educational, Scientific and Cultural Organization (March 2021). The Next Rembrandt. Retrieved from https://en.unesco.org/artificial-intelligence/ethics/cases
- ↑ (March 2021). The Next Rembrandt. Retrieved from https://www.nextrembrandt.com
- ↑ Authors: Yuheng Wu, Yi Mou, Zhipeng Li, Kun Xu, Investigating American and Chinese Subjects’ explicit and implicit perceptions of AI-Generated artistic work, Computers in Human Behavior, Volume 104, 2020, 106186, ISSN 0747-5632, https://doi.org/10.1016/j.chb.2019.106186. Retrieved from: (https://www.sciencedirect.com/science/article/pii/S074756321930398X)
- ↑ Epstein, Ziv, et al. “Who Gets Credit for AI-Generated Art?” IScience, vol. 23, no. 9, Elsevier Inc, 2020, p. 101515, doi:10.1016/j.isci.2020.101515.