Difference between revisions of "Gender bias in the Online Job Search"
(→Job Recruitment Algorithms) |
(→Job Recruitment Algorithms) |
||
Line 9: | Line 9: | ||
For example, in 2018, more than 20 female employees at Fox News complained of sexual harassment, further alleging that these harassment cases were hindering their ability to advance in the workplace. In response, Fox News replaced their hiring process with a machine learning algorithm that analyzed previously successful employees (within the last 21 years) and identified them for potential promotion. However, issues arose when it was brought up that the algorithm would simply perpetuate biases that existed in historical hiring processes. Namely, most employees who had been at the company for a few years, been promoted once or twice, etc., were historically men.<ref name=foxissue> https://www.npr.org/transcripts/580617998 </ref> Automation based on biased data sets simply allows us to repeat our past practices and patterns, thereby automating the status quo. | For example, in 2018, more than 20 female employees at Fox News complained of sexual harassment, further alleging that these harassment cases were hindering their ability to advance in the workplace. In response, Fox News replaced their hiring process with a machine learning algorithm that analyzed previously successful employees (within the last 21 years) and identified them for potential promotion. However, issues arose when it was brought up that the algorithm would simply perpetuate biases that existed in historical hiring processes. Namely, most employees who had been at the company for a few years, been promoted once or twice, etc., were historically men.<ref name=foxissue> https://www.npr.org/transcripts/580617998 </ref> Automation based on biased data sets simply allows us to repeat our past practices and patterns, thereby automating the status quo. | ||
− | Another example of a similar scandal occurred at Amazon in 2018, where a recruiting machine learning algorithm taught itself to penalize female applicants. For example, it downgraded resumes that included the word “women’s,” as in “women’s soccer team captain.” Similarly, it penalized graduates of all women’s colleges, as most historical employees were not graduates of these schools | + | Another example of a similar scandal occurred at Amazon in 2018, where a recruiting machine learning algorithm taught itself to penalize female applicants. For example, it downgraded resumes that included the word “women’s,” as in “women’s soccer team captain.” Similarly, it penalized graduates of all women’s colleges, as most historical employees were not graduates of these schools <ref name=amazon> Dastin, J. (2018, October 10). <i>Amazon scraps secret AI recruiting tool that showed bias against women.</i> Reuters. https://www.reuters.com/article/us-amazon-com-jobs-automation-insight/amazon-scraps-secret-ai-recruiting-tool-that-showed-bias-against-women-idUSKCN1MK08G</ref>. Since this scandal, Amazon has scrapped its recruiting algorithm, but has not publicly addressed the issue or explained how it is handling recruiting efforts moving forward.<ref name=amazonscandal2> https://becominghuman.ai/amazons-sexist-ai-recruiting-tool-how-did-it-go-so-wrong-e3d14816d98e?gi=5e513b501d8b </ref> |
Utilizing real-world data to shape algorithms leads to algorithms producing biased outcomes. Algorithms make predictions by analyzing past data <ref name=paulineKim>Kim, P. T. (2019). Big data and artificial intelligence: New challenges for workplace equality. <i>University of Louisville Law Review</i>, 57(2), 313-328.</ref>. If the past data includes biased judgments, then the algorithm’s predictions will also be biased. Facebook’s tool called “lookalike audience” allows advertisers – in this case, employers – to input a “source audience” that will dictate who Facebook advertises jobs to, based on a person’s similarities with this “source audience” <ref name=paulineKim>Kim, P. T. (2019). Big data and artificial intelligence: New challenges for workplace equality. <i>University of Louisville Law Review</i>, 57(2), 313-328.</ref>. This tool is meant to help employers predict which users are most likely to apply for jobs. If an employer provides the lookalike tool with a dataset that does not include a lot of women, then Facebook will not advertise the job to women. Employers might use this tool to deliberately exclude certain groups, but there is also a possibility for employers to be unaware of the bias of their “source audience” <ref name=paulineKim>Kim, P. T. (2019). Big data and artificial intelligence: New challenges for workplace equality. <i>University of Louisville Law Review</i>, 57(2), 313-328.</ref>. | Utilizing real-world data to shape algorithms leads to algorithms producing biased outcomes. Algorithms make predictions by analyzing past data <ref name=paulineKim>Kim, P. T. (2019). Big data and artificial intelligence: New challenges for workplace equality. <i>University of Louisville Law Review</i>, 57(2), 313-328.</ref>. If the past data includes biased judgments, then the algorithm’s predictions will also be biased. Facebook’s tool called “lookalike audience” allows advertisers – in this case, employers – to input a “source audience” that will dictate who Facebook advertises jobs to, based on a person’s similarities with this “source audience” <ref name=paulineKim>Kim, P. T. (2019). Big data and artificial intelligence: New challenges for workplace equality. <i>University of Louisville Law Review</i>, 57(2), 313-328.</ref>. This tool is meant to help employers predict which users are most likely to apply for jobs. If an employer provides the lookalike tool with a dataset that does not include a lot of women, then Facebook will not advertise the job to women. Employers might use this tool to deliberately exclude certain groups, but there is also a possibility for employers to be unaware of the bias of their “source audience” <ref name=paulineKim>Kim, P. T. (2019). Big data and artificial intelligence: New challenges for workplace equality. <i>University of Louisville Law Review</i>, 57(2), 313-328.</ref>. |
Revision as of 17:08, 25 March 2021
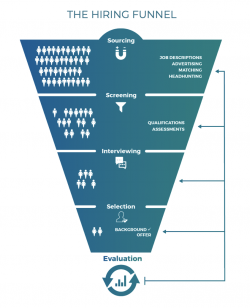
Gender bias refers to the “unfair difference” in the way that both men and women are treated [2]. In the context of the online job search, this refers to the advantapuge male job seekers have versus female job seekers. The advancement of technology and big data has led to companies recruiting and hiring potential employees through the use of big data and artificial intelligence [3]. The use of algorithms in the job search process can perpetuate existing biases which result in ethical implications regarding job opportunities for females being blocked, and the disadvantage for female job seekers due to systematic gender roles.
Evidence of Bias
Job Recruitment Algorithms
Job recruitment algorithms have been found to reinforce and perpetuate unconscious human gender bias [4]. Because job recruitment algorithms are trained on real-world data – and the real world is biased – the algorithms amplify this bias on a larger scale. Decision-making algorithms are “designed to mimic how a human would…choose a potential employee” and without careful consideration, algorithms can intensify bias in recruiting [5].
For example, in 2018, more than 20 female employees at Fox News complained of sexual harassment, further alleging that these harassment cases were hindering their ability to advance in the workplace. In response, Fox News replaced their hiring process with a machine learning algorithm that analyzed previously successful employees (within the last 21 years) and identified them for potential promotion. However, issues arose when it was brought up that the algorithm would simply perpetuate biases that existed in historical hiring processes. Namely, most employees who had been at the company for a few years, been promoted once or twice, etc., were historically men.[6] Automation based on biased data sets simply allows us to repeat our past practices and patterns, thereby automating the status quo.
Another example of a similar scandal occurred at Amazon in 2018, where a recruiting machine learning algorithm taught itself to penalize female applicants. For example, it downgraded resumes that included the word “women’s,” as in “women’s soccer team captain.” Similarly, it penalized graduates of all women’s colleges, as most historical employees were not graduates of these schools [7]. Since this scandal, Amazon has scrapped its recruiting algorithm, but has not publicly addressed the issue or explained how it is handling recruiting efforts moving forward.[8]
Utilizing real-world data to shape algorithms leads to algorithms producing biased outcomes. Algorithms make predictions by analyzing past data [3]. If the past data includes biased judgments, then the algorithm’s predictions will also be biased. Facebook’s tool called “lookalike audience” allows advertisers – in this case, employers – to input a “source audience” that will dictate who Facebook advertises jobs to, based on a person’s similarities with this “source audience” [3]. This tool is meant to help employers predict which users are most likely to apply for jobs. If an employer provides the lookalike tool with a dataset that does not include a lot of women, then Facebook will not advertise the job to women. Employers might use this tool to deliberately exclude certain groups, but there is also a possibility for employers to be unaware of the bias of their “source audience” [3].
Job Recommendation Algorithms
Job recommendation algorithms within online platforms are built to find and reproduce patterns in user behavior, updating predictions or decisions as job seekers and employers interact [9]. If the system within the platform recognizes that the employer interacts with mostly men, then the algorithm will look for those characteristics in potential job applicants and replicate the pattern [9]. This pattern picked up by the algorithm can happen without specific instruction from the employer, which leads to biases going unnoticed.
Algorithms Extending Human Bias
Personal, human bias bleeds into algorithmic bias. A study conducted at the University of California, Santa Barbara found that people’s own underlying biases were bigger determinants of their likelihood to apply to jobs than any gendered job posting [10]. This underlying human bias needs to be reduced in order to work towards gender neutrality in the job market [10]. Humans choose the data to train algorithms with, and the "choice to use certain data inputs over others can lead to discriminatory outcomes" [11]. Hiring algorithms can be an extension of "our opinions embedded in code" and further research highlights that algorithms reproduce existing societal, human bias [11]. The people constructing hiring algorithms are in the tech industry, which is not diverse, leading to the algorithms being trained on non-diverse data, and therefore extending human gender bias into the online job market [11]. Unconscious human bias can contribute to algorithms being biased, as the human biases will be built into the algorithms.
Ethical Implications
Algorithms Blocking Opportunities
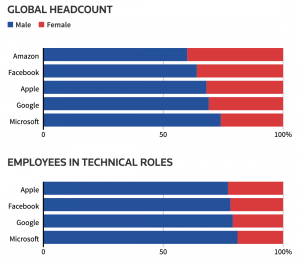
Algorithms in the online job search do not outright reject job seekers, they simply do not show certain groups of job seekers opportunities they are qualified for; as legal scholar Pauline Kim stated, “not informing people of a job opportunity is a highly effective barrier” to job seekers [9]. Qualified candidates cannot apply for a job if they have not been shown the opportunity.
Amazon’s algorithmic recruiting tool was trained with 10 years’ worth of resumes that were sent to Amazon; however, because technology is a male-dominated field, most of the resumes were from male applicants, leading the algorithm to downvote women [7]. This method of algorithm training taught the algorithm that men were more preferable, and it would penalize a candidate who included the word “women’s” in their resume, for example, if they listed an activity as “women’s team captain” [7].
Traditional Gender Roles Affect Outcomes
It has been studied that women can have a better keyword match on their resume, yet not be selected if a man has more experience than them [5]. These hiring algorithms built and trained by humans do not take into account the time women have to take off of work to have children or take care of children [5]. The author of a study done by the University of Melbourne recounts that “women have less experience because they take time [off work] for caregiving, and that algorithm is going to bump men up and women down based on experience” [4]. Because women are more likely to experience a disruption in their career due to children, they will be viewed as a lesser candidate by the algorithm, even if they have more relevant experience than a male candidate [5]. Hiring algorithms do not take into account gender roles, and women taking time off to give birth, which replicates and reinforces gender bias in the hiring process [5].
Reducing Bias
Rethinking How Algorithms are Built
Vendors that are building the recruitment algorithms targeting specific job seekers need to think beyond minimum compliance requirements and have to consider whether or not the algorithm they are building is leading to more fair hiring outcomes [9]. Additionally, the people stating that their algorithms will reduce bias in the hiring process have to build and test their algorithms while keeping that goal in mind, or else the technology will continue to undermine the online job search process [9]. Re-thinking algorithms and how to build them will begin to reduce bias in the job search, as many factors need to be considered. An additional point of contention in the algorithmic justice space is diversifying the engineers who are working on building these algorithms. For example, in 2015, Google Photos user Jacky Alcine posted a viral tweet that displayed Google Photos' facial recognition software classifying a photo of him and his friend as gorillas [12]. This raised the question: would such an oversight in Google's training data have occurred if there were more Black engineers at the company? We would likely see significant reduction in algorithmic biases with an increase in diversity in the technology field.
Balancing Humans and Algorithms
Implementing a balance between predictive algorithms and human insight is a promising solution for employers looking to use algorithms in their hiring process while reducing bias [11]. Using artificial intelligence and algorithms to parse through large amounts of data or applicants works well for processing. Balancing the processing by algorithms with the "human ability to recognize more intangible realities of what that data might mean" is the second step in the process of limiting algorithmic bias [11]. For a partnership between humans and algorithms to be successful within companies, they need to consciously and deliberately implement new practices [11]. Both algorithms and humans still need to be held accountable for reducing bias, and working together would encourage a good short-term solution to the phenomenon of gender bias in the online job search [11].
References
- ↑ Bogen, M. & Rieke, A. (2018). Help Wanted: An Examination of Hiring Algorithms, Equity, and Bias. Upturn. https://www.upturn.org/static/reports/2018/hiring-algorithms/files/Upturn%20--%20Help%20Wanted%20-%20An%20Exploration%20of%20Hiring%20Algorithms,%20Equity%20and%20Bias.pdf
- ↑ Cambridge Dictionary.(n.d.). Gender Bias. In Cambridge English Dictionary. https://dictionary.cambridge.org/us/dictionary/english/gender-bias
- ↑ 3.0 3.1 3.2 3.3 Kim, P. T. (2019). Big data and artificial intelligence: New challenges for workplace equality. University of Louisville Law Review, 57(2), 313-328.
- ↑ 4.0 4.1 Hanrahan, C. (2020, December 2. Job recruitment algorithms can amplify unconscious bias favouring men, new research finds. The ABC News. https://www.abc.net.au/news/2020-12-02/job-recruitment-algorithms-can-have-bias-against-women/12938870
- ↑ 5.0 5.1 5.2 5.3 5.4 Cheong, M., et al. (n.d.). Ethical Implications of AI Bias as a Result of Workforce Gender Imbalance. The University of Melbourne. https://about.unimelb.edu.au/__data/assets/pdf_file/0024/186252/NEW-RESEARCH-REPORT-Ethical-Implications-of-AI-Bias-as-a-Result-of-Workforce-Gender-Imbalance-UniMelb,-UniBank.pdf
- ↑ https://www.npr.org/transcripts/580617998
- ↑ 7.0 7.1 7.2 7.3 Dastin, J. (2018, October 10). Amazon scraps secret AI recruiting tool that showed bias against women. Reuters. https://www.reuters.com/article/us-amazon-com-jobs-automation-insight/amazon-scraps-secret-ai-recruiting-tool-that-showed-bias-against-women-idUSKCN1MK08G
- ↑ https://becominghuman.ai/amazons-sexist-ai-recruiting-tool-how-did-it-go-so-wrong-e3d14816d98e?gi=5e513b501d8b
- ↑ 9.0 9.1 9.2 9.3 9.4 Bogen, M. (2019, May 6). All the Ways Hiring Algorithms Can Introduce Bias. Harvard Business Review. https://hbr.org/2019/05/all-the-ways-hiring-algorithms-can-introduce-bias
- ↑ 10.0 10.1 Tang, S., et al. (2017). Gender Bias in the Job Market: A Longitudinal Analysis. ACM on the Human-Computer Interaction. . https://dl.acm.org/doi/epdf/10.1145/3134734
- ↑ 11.0 11.1 11.2 11.3 11.4 11.5 11.6 Raub, M. (2018). Bots, Bias and Big Data: Artificial Intelligence, Algorithmic Bias and Disparate Impact Liability in Hiring Practices. Arkansas Law Review, 71(2), 529-570
- ↑ Cite error: Invalid
<ref>
tag; no text was provided for refs namedgooglephotosscandal