Difference between revisions of "Bias in Information"
Line 106: | Line 106: | ||
==== Echo Chambers ==== | ==== Echo Chambers ==== | ||
− | Similar to filter bubbles, echo chambers are the result of filtering and personalization algorithms. Echo chambers are described as, "a situation where certain ideas, beliefs or data points are reinforced through repetition of a closed system that does not allow for the free movement of alternative or competing ideas or concepts."<ref>[[https://www.techopedia.com/definition/23423/echo-chamber Echo Chambers]], Technopedia. Retrieved 17 April 2019.</ref> | + | Similar to filter bubbles, echo chambers are the result of filtering and personalization algorithms. Echo chambers are described as, "a situation where certain ideas, beliefs or data points are reinforced through repetition of a closed system that does not allow for the free movement of alternative or competing ideas or concepts."<ref>[[https://www.techopedia.com/definition/23423/echo-chamber Echo Chambers]], Technopedia. Retrieved 17 April 2019.</ref> The result of echo chambers is a system in which alternate ideas and concepts are never introduced and the ideals of the system are "echoed" back and forth without a change in discourse. Echo chambers are primarily found on social media platforms and often times form surrounding political ideologies. |
== References == | == References == |
Revision as of 20:22, 17 April 2019
Bias in information is recognized when searches for information produce differing results, and sequentially produce different interpretations of those results. When a user is searching for information they are searching for “the resolution of uncertainty” [1]. The information that is provided to the searcher, in conjunction with the searcher’s understanding of that information, can lead to discrepancies in the searcher's knowledge on the topic. The act of filtering results in a specific way or only allowing certain information to be accessible to an observer can drastically change the value and meaning of the content provided. Online search engines, as a product of the computer revolution, provide a space for bias in information to exist. The prevalence of bias in information, especially among search engines, has lead to ethical concerns regarding privacy, the filtering of search results, and the types of biases that may occur among social groups.
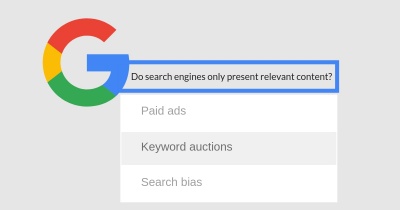
Contents
Type of Bias
There exists many different types of bias in information which can be put into three main sections consisting of general bias, research bias, and news bias. Among the types of bias in general bias are confirmation bias and groupthink/bandgwagon bias. Research bias consists of selection bias and anchoring bias. The types of news bias include commercial bias, bad news bias, status quo bias, access bias, visual bias, fairness bias, and narrative bias. All of these different bring about bias in information in a variety of different ways. These types are described here.
General Bias
Confirmation Bias
Confirmation bias is the tendency for users to interpret new evidence or information as confirmation to their current beliefs. This bias is seen very commonly in sites online, particularly media sites, where users and publishers only present information that backs up their arguments or points. [3] Confirmation bias has the potential to lead to self-fulfilling modes of thinking that may inhibit civil discussion or debate.
Groupthink/Bandwagon Bias
Groupthink or Bandwagon bias may occur in settings where large groups of people share a common motive for coming together. Out of fear of becoming isolated from the group, participants will typically try to maintain a harmonious work environment and will refrain from sharing their honest opinions on controversial decisions and opinions made among the group. [3] Groupthink/Bandwagon bias also limits the amount of public civil discussion and debate as participants are reluctant to oppose the decisions of the group.
Research Bias
Selection Bias
Selection bias is a bias that occurs most commonly in research, where researchers pick the number of users and the type of users who are being used for research. This results in non-random participants, which makes it nearly impossible to validate the actual findings found in the research. [3] Researchers are able to tailor the participants picked in a way that yields results in line with their bias.
Anchoring Bias
Anchoring bias occurs when users or researchers use one single piece of information to make subsequent decisions. In reality there should be a number of pieces of information which researchers use in order to make subsequent decisions which would avoid bias. Once an Anchor is set, users will continue to base all actions and decisions based off of that anchor and it is particularly difficult to remove once established. [3]
News Bias
Commercial Bias
Commercial bias simply means that the news must remain "new," so news outlets rarely ever follow up on stories that have already been reported on or stories that are considered to be "old." This leads to a bias in the information being reported on as always some sort of "new" content. [3]
Bad News Bias
Bad news bias is when news outlets highlight stories that are scary or threatening because it generates more views. News providers attempt to peak the interest of viewers with these shocking stories in order to benefit themselves. This creates a bias in the types of stories that are reported on as generally less scary and threatening stories yields lower viewership and thus aren't shown as often. [3]
Status Quo Bias
Status quo bias is the preference people have for things to stay the same, which causes new outlets to never really change the way that they report on things. This type of bias generally stems from people fearing the consequences of changing their preferences to something "new."[4] News outlets exhibit status quo bias by reporting on the same types of stories in fear of losing viewership if they change the stories which they report on.
Access Bias
Journalists and readers may compromise the transparency of the news in order to get access to powerful people as story sources. News outlets then create a bias in the information they report on as they are simply leveraging the power of well-known public figures.
Visual Bias
Stories with a visual hook are more likely to get noticed than those without a visual hook. News outlets generally will report on stories that have some sort of visual appear to their audience as news with no visual aspect will likely get little attention.[5] This leads to a bias in the types of stories and information which news outlets report on as they are reporting more on stories with visual aspect that will bring in a larger audience viewership.
Fairness Bias
Fairness bias is when reporters present opposing viewpoints in order to be "fair", even when they are not equally valid as the opposing viewpoint may not be contentious. This bias is most prevalent in news reporting on politics. [5] News outlets seek to create the idea that politicians are always in opposition and never agree. This can lead to bias in which news outlets are attacking one party or another.
Narrative Bias
News outlets present a story to be like a narrative, with a beginning, middle, and an ending. However, many real-life news stories are middle, and there shouldn't be an ending including in the news report. Journalists try to tackle the problem by inserting a provisional ending, therefore making the reports seem more conclusive than they actually are. This type of bias in news reporting attempts to create drama through the narrative story line as it generally leads to more interesting stories and thus higher viewership.[5]
Expediency Bias
Expediency bias is when news outlets seek to report on information that can be obtained quickly, easily, and inexpensively.[5] News outlets are extremely competitive and seek to report on the most immediate information in order to gain higher viewership. This leads to bias in the information reported on being the quick and easy information rather than reporters taking their time to get better sources and craft a more well-rounded story.
Glory Bias
Glory bias is prevalent when news reporters assert themselves into the story which they are reporting on. [5] This type of bias leads to journalists attempting to establish a cultural identity as a knowledgeable insider. In reality journalists should remain as an outside observer to their stories and merely report on them in an unbiased manner.
Search Engine Results
The First 10 results
A search engine provides thousands of results as a list of ranked items where the top items are considered to be the most "important" or "relevant" pages according to the user's given query. When researching a specific topic, if the first few results don’t provide the user with what he or she was searching for, this individual may repeatedly refine the contents of the query until they find exactly what they are looking for. As a result, the first few links that appear when a user performs their search may repeatedly be ranked highest among the returned results from query to query, and thus may exclude a number of important, potentially opposing pieces of content. An example being Safiya Umoja Noble's research of the misrepresentative results given after searching the term "black girls".[6] In the context of news and media, this may lead to a number of self-fulfilling biases and or discriminatory biases.
Information Overload
Information overload is just what it sounds like, it is quite literally that there is too much information. The amount of information readily available to the public has only increased, with the rise of technology, to the point that a user can be provided with too much information [7]. Information overload is exhibited through the thousands of results given by search engines and can make it seemingly impossible for an average user to parse through all of the information that exists.
Information overload predates the era of modern technology. It can be seen in older scenarios as well. For example, in both libraries and museums, we see overload as one human could not possibly read all of the books in an extensive library or fully study all aspects of a museum. Excessive information can prevent a user from understanding certain information which further may prevent them from making an informed decision.
Search Engines
A search engine is a software system that is designed to carry out a web search on a particular query or phrase that is provided by a user. The information provided from a search can include many different types of media some of which include: articles, documents, images, videos, and infographics. Search engines provide easy access to information that can also be available in specific locations like libraries and museums. Search engines are the most common form of finding information today. Google, for example, process 40,000 queries a second [8], which accounts to 3.5 billion searches a day and 1.2 trillion searches a year.
How Search Engines Work
A search engine is able to provide thousands of results in second and is able to do so because of the work that occurs in the background. In the background, there are three major steps: web crawling, indexing, and the algorithm the search engine performs[9]. In the first step, a web crawler searches the World Wide Web in order to find specific documents to add to the search engine’s personal collection. Every time a document is updated or a new document is found, a crawler will add a copy of this document to a collection. This collection of documents, now kept by the search engine in a data center, can be organized and searched through based off of what a user is looking for. In the last step, the algorithm, a search engine must decide how to organize the documents to provide the user with a ranked set of results where ideally the first thing the user sees is what is most relevant to the user’s search. The ranking of these results is based conceptually on how many connections a result has to other potential results. This ranking protocol is referred to as PageRank. [10] Before these three steps can occur, however, a user must write a query for the technology to compute results for. Typically we see this as a phrase, but can also be any type of media, for example, a picture.
Search Engine Optimization (SEO)
Search Engine Optimization refers to the process of optimizing a website or web page's online visibility from a web search through organic search results. SEO is all about attempting to improve search engine rankings, especially on Google. SEO is a major internet marketing strategy and is examined to detect the most useful keywords and search terms typed into search engines in order to attempt to produce a higher ranked result for a given website.
Ethical Concerns
The search for information is an inevitable process which causes many ethical concerns to arise. These ethical concerns come from the bias involved in the search engine design, the filtering of results, and the privacy of the user.
Privacy
Along with the process of finding optimal results, a search engine will also track certain information about a user behind the scenes. The time and date, along with the content, of each query that is searched along with the IP address of the computer searching it is all information that is stored. Although unlikely, pooling similar IP address can get a list of searches by a specific user[11]. The IP address shared with the search engine are not of personal computers but instead of your local router. This gives specific information on geolocation and the types of searches that occur in specific locations. This use of address can be used against users in specific scenarios, for example in China the use of google is prohibited and instead provides different search engines for the country.[12]
Along with the ability to ban specific phrases in certain locations, a search engine also uses past searches and the documents looked as part of their algorithms. When a document is looked at frequently it will move higher up on the list of results due to the fact that users find it relevant. Many websites such as Youtube and Netflix adopt the recommender system that conducts personalized information filtering using search and view history or tracking cookies. The methods that the companies use to gather data are problematic because in most cases users are uninformed, and even so the notifications used to ask for users' consent are sometimes too vague or hard for users to understand.[13]
Bias
Due to the nature of a search engine, and the processes it goes through to provide results, bias can be introduced into the process in each step. In “Values in technology and disclosive computer ethics”, Brey discusses the idea that technology has “embedded values” which means that computers and their software are not “morally neutral” [14]. Somewhere in the process of their design, computers can favor specific values.
Brey discusses three types of biases which we can use to relate and define in search engines :
- Preexisting Bias
- Technical Bias
- Emergent Bias
The first, preexisting bias, occurs when values and attitudes exist prior to the development of the software. In our breakdown of software systems, we can see this when an order of documents is provided after a search. If the algorithm of the system always favors certain documents over others, without any interference from outside sponsors, we might always receive the documents first that reflect the values of the creator of the algorithm.
The second bias, technical bias, occurs due to the limitations of the software. Due to the nature of search engines, and the way that humans use them, where often only the first results are even looked at, it is impossible to display certain results – or for humans to even see certain results. Taking it a step further, the documents that can be gathered also have certain limitations. Only the information that is available can be crawled upon and added to the collection. In many situations, the information provided can lead to bias, due to the fact that there might be more information for specific things than others.
The last, emergent bias occurs when the system is being used in a way not intended by its designers. When a user enters a phrase, the wording of the phrase can be very important. Different words with the same meaning often have different connotations that can provide different results.
Social Bias
Studies show search engines reinforce many social biases and stereotypes. In particular, Google images has been criticized for its lack of diversity in some search results [3]. For example, when searching the word “doctor” there are significantly more men than women pictured. Although there are more male doctors than female doctors, Google images shows a very disproportionate amount of male to female doctors. Another report showed when searching three black teenagers Google images shows a series of mugshot photos, where as when searching three white teenagers images of smiling young adults appear [3]. Google search results are affected by preexisting bias, technical bias, and emergent bias. This is harmful because these results perpetuate societal stereotypes and values. Engineers and users must be mindful of the implications of these biases and work to overcome them in technology and society.
Filtering Results
Showing results to an individual is a process that is dealt with by a search engine's algorithm. Finding the most relevant documents is done so by uniquely identifying and categorizing documents based off of their subject. Due to the nature of receiving a list of relevant documents, it is impossible for all of the results to be shown at once or even seen. Knowing this, search results can be influenced by advertisements and specific companies or sites sponsoring their own documents to be shown above others. Certain documents then receive unfair advantages to be shown higher up than others and can influence the information that will be viewed.
Customized information filtering not only poses concerns to privacy issues but also limit the information that users get exposed to. For instance, Netflix's recommender system gives priorities to information similar to what users have searched or viewed previously. As Xavier Amatriain, the Director of Algorithms Engineering at NetflixFor, says, "over 75% of what people watch comes from our commendations."[15] It is very likely that the similarities among the information can trap users in a loop that isolates them from having the choice of accessing new information. [13]
Filter Bubbles
Search engine's and other services which make use of filtering algorithms in order to tailor user's results towards their interests end up creating what are known as "filter bubbles." Eli Pariser describes these search engines as "creating a unique universe of information for each of us." [16] Pariser coined the term filter bubble to describe this unique universe of information. He believes that filter bubbles present three new dynamics to personalization:[16]
- 1. Each user is alone in their filter bubble.
- 2. The filter bubble is invisible.
- 3. Each user doesn't choose to enter their prospective filter bubble.
The implications of this are that every user who uses the filtering mechanisms of search engines or other services is trapped in their own filter bubble of personalization unknowingly. These users don't choose to enter the filter bubbles, however search algorithms are able to create the filter bubbles for them by using the user's search history.[16]
Filter bubbles provide the benefit of creating a personalized universe of information for each user, however also yields some negative consequences. As the filter bubble is created it only shows users things that are similar to their previous interests.[16] This can lead to users confining their interests to what they know they like and restricting them from trying content which is new or different from their interests. It results in users not being able to branch out and explore other content.[16]
Filter bubbles also grow smaller and more precise over time. As filtering and search algorithms gather more data on their users, they are able to provide more accurate recommendations which in turn leads to more restricted search results. As the filter bubble grows smaller, the amount of unique content which each user sees diminishes as well.[16]
Echo Chambers
Similar to filter bubbles, echo chambers are the result of filtering and personalization algorithms. Echo chambers are described as, "a situation where certain ideas, beliefs or data points are reinforced through repetition of a closed system that does not allow for the free movement of alternative or competing ideas or concepts."[17] The result of echo chambers is a system in which alternate ideas and concepts are never introduced and the ideals of the system are "echoed" back and forth without a change in discourse. Echo chambers are primarily found on social media platforms and often times form surrounding political ideologies.
References
- ↑ “Information.” Wikipedia, Wikimedia Foundation, 9 Apr. 2019, en.wikipedia.org/wiki/Information.
- ↑ “Should the Google Search Engine Be Answerable To Competition Regulation Authorities?” Economic and Political Weekly, 7 Sept. 2018, www.epw.in/engage/article/should-google-search-engine-be.
- ↑ 3.0 3.1 3.2 3.3 3.4 3.5 3.6 3.7 Ching, Teo Choong, and Teo Choong Ching. “Types of Cognitive Biases You Need to Be Aware of as a Researcher.” UX Collective, UX Collective, 27 Sept. 2016, uxdesign.cc/cognitive-biases-you-need-to-be-familiar-with-as-a-researcher-c482c9ee1d49.
- ↑ [Status quo bias] behaviorialeconomics.com. Retrieved 15 April 2019.
- ↑ 5.0 5.1 5.2 5.3 5.4 [Rhetorica], Media/Political Bias. Retrieved 15 April 2019.
- ↑ Noble, Safiya Umoja. “Critical Surveillance Literacy in Social Media: Interrogating Black Death and Dying Online.” Black Camera, vol. 9, no. 2, 2018, p. 147., doi:10.2979/blackcamera.9.2.10.
- ↑ “Information Overload.” Wikipedia, Wikimedia Foundation, 28 Mar. 2019, en.wikipedia.org/wiki/Information_overload.
- ↑ “Google Search Statistics.” Google Search Statistics - Internet Live Stats, www.internetlivestats.com/google-search-statistics/.
- ↑ “How Do Search Engines Work? - BBC Bitesize.” BBC News, BBC, 23 Oct. 2018, www.bbc.com/bitesize/articles/ztbjq6f.
- ↑ “PageRank.” Wikipedia, Wikimedia Foundation, 10 Apr. 2019, en.wikipedia.org/wiki/PageRank.
- ↑ Weissman, Cale Guthrie. “What Is an IP Address and What Can It Reveal about You?” Business Insider, Business Insider, 18 May 2015, www.businessinsider.com/ip-address-what-they-can-reveal-about-you-2015-5.
- ↑ Replacement of Google with Alternative Search Systems in China - Documentation and Screen Shots, cyber.harvard.edu/filtering/china/google-replacements/.
- ↑ 13.0 13.1 Fröding, Barbro, and Martin Peterson. “Why Virtual Friendship Is No Genuine Friendship.” SpringerLink, Springer Netherlands, 6 Jan. 2012, link.springer.com/article/10.1007/s10676-011-9284-4.
- ↑ Brey, Philip. “Values in Technology and Disclosive Computer Ethics (Chapter 3) - The Cambridge Handbook of Information and Computer Ethics.” Cambridge Core, Cambridge University Press, www.cambridge.org/core/books/cambridge-handbook-of-information-and-computer-ethics/values-in-technology-and-disclosive-computer-ethics/4732B8AD60561EC8C171984E2F590C49.
- ↑ 15.0 15.1 Amatriain, Xavier. “Machine Learning & Recommender Systems at Netflix Scale.” InfoQ, InfoQ, 16 Jan. 2014, www.infoq.com/presentations/machine-learning-netflix.
- ↑ 16.0 16.1 16.2 16.3 16.4 16.5 Pariser, Eli. The Filter Bubble: What the Internet is Hiding From You. The Penguin Press, New York, 2011. Retrieved 17 April 2019.
- ↑ [Echo Chambers], Technopedia. Retrieved 17 April 2019.