Difference between revisions of "Automated Decision Making in Child Protection"
m |
m |
||
(23 intermediate revisions by 5 users not shown) | |||
Line 1: | Line 1: | ||
− | Automated decision-making regarding child protection refers to | + | '''Automated decision-making regarding [https://en.wikipedia.org/wiki/Child_protection child protection]''' refers to using software in an attempt to quantify the future possibility of harm for a child in a given context. This type of program is used along with caseworkers as a method of screening calls placed about possible domestic violence situations to determine the risk level of a child involved.<ref name="PA">Russell, J. (2015). Predictive analytics and child protection: Constraints and opportunities. <i>Child Abuse & Neglect, 46</i>, 182-189. https://doi.org/10.1016/j.chiabu.2015.05.022</ref> In the United States, this system has been deployed in Allegheny County, Pennsylvania, with some mixed results.<ref name="chouldechova">Chouldechova, A. (2018). A case study of algorithm-assisted decision making in child maltreatment hotline screening decisions. <i>Conference on Fairness, Accountability, and Transparency, 81</i>(1). Retrieved April 10, 2021, from http://proceedings.mlr.press/v81/chouldechova18a/chouldechova18a.pdf</ref> Child protection decision-making software is an application of [https://en.wikipedia.org/wiki/Predictive_analytics predictive analytics], and some argue that it carries ethical concerns, some of which are unique to this specific application.<ref name="PA"/> |
− | = Functionality = | + | ==Functionality== |
+ | The system's goal is to apply various forms of trend identification to predict the likelihood of an event occurring in the future that could endanger a child.<ref name="coding">Glaberson, S. K. (2019). Coding Over the Cracks: Predictive Analytics and Child Protection. <i>Fordham Urban Law Journal, 46</i>(2), 306-363. Retrieved April 2, 2021, from https://ir.lawnet.fordham.edu/ulj/vol46/iss2/3</ref> New predictive tools take in vast amounts of administrative data and weigh each variable to identify situations that warrant intervention, and some are working to expose potential sources of bias and unfairness in these tools.<ref name="AJ">Keddell, E. (2019). Algorithmic Justice in Child Protection: Statistical Fairness, Social Justice and the Implications for Practice. <i>Social Sciences, 8</i>(10), 281. https://doi.org/10.3390/socsci8100281</ref> | ||
− | + | Childhood protection algorithms vary from model to model, but often, as Stephanie Glaberson describes, these predictive analysis techniques use [https://en.wikipedia.org/wiki/Machine_learning machine learning] strategies to assess data and create the models. Glaberson describes this process as a product of the curated data and the programmers developing the machine learning algorithms. For example, it requires the developers to choose problem areas or factors for the predictive techniques to target and take into consideration.<ref name="coding"/> These algorithms are critically described by some as "black box" algorithms, meaning the details and specifics are unclear, especially to those who are using the algorithm and were not involved in its creation.<ref name="coding"/><ref name="AJ"/><ref>Merriam-Webster. (n.d.). Black Box. In <i>Merriam-Webster.com dictionary</i>. Retrieved April 10, 2021, from https://www.merriam-webster.com/dictionary/black%20box</ref> | |
− | + | ==Examples== | |
+ | ===Pennsylvania=== | ||
+ | [[File:PAScreenTool.jpeg|thumbnail|350px|right|Photo of Allegheny Family Screening Tool on a desktop computer. Casey Family Programs. https://www.casey.org/hope2018/]] | ||
+ | In August of 2016, Pennsylvania’s Allegheny County became the first in the United States to use automated decision-making software, the Allegheny Family Screening Tool (AFST), to supplement its existing child abuse hotline screening process. The tool offers a risk score as a “second opinion” to the call operator’s initial verdict, allowing them to change their mind over whether or not to flag the call as worthy of an investigation.<ref name="danger">Hurley, D. (2018, January 2). <i>Can An Algorithm Tell When Kids Are in Danger?</i> The New York Times. https://www.nytimes.com/2018/01/02/magazine/can-an-algorithm-tell-when-kids-are-in-danger.html</ref> Flagging the call as worthy of investigation is referred to as "screening in," whereas offering outside resources and opting for no investigation is called "screening out."<ref name="county">Allegheny County. (n.d.). <i>The Allegheny Family Screening Tool</i>. https://www.alleghenycounty.us/Human-Services/News-Events/Accomplishments/Allegheny-Family-Screening-Tool.aspx</ref> This risk score provided by the AFST is based on two factors: re-referral (the likelihood that, upon a "screen out," the child or children will be referred back within two years) and placement (the likelihood that, upon a "screen in," the child or children will be removed from their home within two years). | ||
− | + | A case study by Chouldechova et al. found the AFST to have implementation mistakes. Errors with the training and testing of the algorithm that created the models resulted in an overestimate of the model's performance results. In April of 2017, the research team began rebuilding the model to correct for these errors.<ref name="chouldechova"/> The AFST, along with other predictive analysis tools relating to child protection, has been criticized by some for the potential of bias or overcompensation.<ref name="danger"/> However, supporters argue that it is not meant to replace human judgment, but to assist.<ref name="county"/> | |
− | + | ===Florida=== | |
+ | In Florida, a non-profit organization called Eckerd Connects (formerly Eckerd Kids), in partnership with a for-profit company called Mindshare Technology, offers its own predictive analytics tool for child protection called Rapid Safety Feedback (RSF). This tool was more widely criticized than AFST due to high risk predictions and reportedly missing children that were high risk but not detected by RSF.<ref name="coding"/><ref name="AJ"/> Further critiques came against Eckerd Connect and RSF after they refused to reveal details about their algorithm, even after the deaths of two children that had not been designated high risk by the RSF tool.<ref name="danger"/> There are five states currently working with Eckerd Connects to adopt the RSF tool in their child welfare systems.<ref>Eckerd Connects. <i>Eckerd Rapid Safety Feedback.</i> Retrieved April 11, 2021, from https://eckerd.org/family-children-services/ersf/</ref> | ||
− | + | ===United Kingdom=== | |
+ | In the United Kingdom, some local councils have turned to algorithmic profiling to predict child abuse; these algorithms are designed to help take pressure off caseworkers and allow them to focus human resources elsewhere.<ref>McIntyre, N., & Pegg, D. (2018, September 16). <i>Councils use 377,000 people's data in efforts to predict child abuse.</i> Retrieved April 2, 2021, from https://www.theguardian.com/society/2018/sep/16/councils-use-377000-peoples-data-in-efforts-to-predict-child-abuse</ref> There are several reasons these councils have turned to technology in recent years. The first is pressure from 2014 media reports of failures within child protection systems.<ref name="AJ"/> The reports were based on a study that found that human caseworkers in the UK were committing three kinds of errors. Slow revision of initial judgments even in light of new evidence, confirmation bias, and witnesses bias (for example, doctors carried more weight than neighbors).<ref name="coding"/> The councils believe that the algorithms may provide an unbiased opinion on which cases to investigate first. Additionally, budget cuts to welfare programs are the primary cause of departments turning to algorithms to assist a reduced number of available caseworkers. In some cases, there are no alternatives for high-quality child maltreatment centers. | ||
− | + | These algorithms collect data on school attendance, housing association repairs, police records on antisocial behavior and domestic violence. Although many believe this data would help the algorithms, critics question using children's sensitive personal data without explicit permission. Data handling is a concern for many citizens in the UK as some believe the Data Protection Act is not equipped to handle data of this magnitude. Most councils are simply passing along alerting information to caseworkers, including school expulsion or domestic violence reports in a child's home. These risk alerts are intended to help caseworkers predict child abuse before it escalates. However, experts wonder if intervention in family life is beneficial in most situations and the risk of false positives impeding a family dynamic. <ref>Brown, P., Gilbert, R., Pearson, R., Feder, G., Fletcher, C., Stein, M., Shaw, T., Simmonds, J. (2018, September 19). <i>Don't trust algorithms to predict child-abuse risk | letters</i>. Retrieved April 2, 2021, from https://www.theguardian.com/technology/2018/sep/19/dont-trust-algorithms-to-predict-child-abuse-risk</ref> | |
− | + | ===The Sweetie Project=== | |
+ | In 2013, the children’s rights organization Terre des Hommes launched a fake digital child called [https://en.wikipedia.org/wiki/Sweetie_(internet_avatar) Sweetie], in an effort to use [https://en.wikipedia.org/wiki/Artificial_intelligence artificial intelligence] to catch online child sex predators. This endeavor used a simulation of a child, completely rendered by computer graphics, named Sweetie. The current model of Sweetie 2.0 uses an automated chat function. It tracked, identified, and threatened users of the offense they were participating in. Sweetie 1.0 required investigators to participate in the chatroom dialogue in order to catch offenders. This allowed human eyes to effectively identify child abuse and take action. It also limited Sweetie’s ability to catch as many offenders as possible. With an automated chat function, Sweetie could track and catch significantly more offenders of virtual child abuse in sex tourism.<ref name="hof">van der Hof, S., Georgieva, I., Schermer, B., Koops, B.-J. (Eds.). (2019). <i>Sweetie 2.0: Using artificial intelligence to fight webcam child sex tourism [Abstract].</i> Asser Press. https://www.springer.com/gp/book/9789462652873</ref> Despite initial reports that Sweetie would no longer be used, in 2019 the work was revived in a project known as #Sweetie 24/7.<ref>Crawford, A. (2013, November 5). <i>Computer-generated 'Sweetie' catches online predators.</i> BBC News. https://www.bbc.com/news/uk-24818769</ref><ref>Terre des hommes. (n.d.). <i>Sweetie, our weapon against child webcam sex</i>. https://www.terredeshommes.nl/en/programs/sweetie</ref> | ||
− | + | ====Controversy==== | |
+ | The Sweetie Project has brought up ethical controversy about the legality of this practice of catching online pedophiles. Criminal laws on the degree of offense of online sex involving a child are unclear, especially when participants are from different countries. Professor Simone van der Hof also explores the question of whether the Sweetie technology constitutes entrapment. Since Sweetie is not yet an official tool for criminal prosecution, the validity of this automated technology for child abuse tracking is debatable.<ref name="hof"/> | ||
− | + | =Morality Concerns= | |
− | + | At least three major ethical concerns exist in applying predictive analytics to child protection.<ref name="silver">Church, C.E. & Fairchild, A.J. (2017). In Search of a Silver Bullet: Child Welfare's Embrace of Predictive Analytics. <i>Juvenile & Family Court Journal, 68</i>(1), 67-81. https://doi-org.proxy.lib.umich.edu/10.1111/jfcj.12086</ref>. The first is a general concern regarding algorithms that perform machine learning, known as [https://en.wikipedia.org/wiki/Algorithmic_transparency algorithmic transpaency]. Algorithmic transparency refers to a program producing results that cannot be [https://en.wikipedia.org/wiki/Reverse_engineering reverse-engineered] due to a lack of information about the algorithm. Missing details about an algorithm's inner workings can result from proprietary software or the nature of machine learning. | |
− | + | ||
− | + | Another concern with predictive learning in child protection is the prediction of rare events. Critical incidents where the abuse of a child could be fatal are near 2% of all events, which leads to an ethical dilemma of preferring [https://en.wikipedia.org/wiki/False_positives_and_false_negatives false positives] or negatives. An algorithm will never be perfect, which leads to the moral question of whether software should return false positives at the cost of resources or false negatives at the price of child safety.<ref name="silver"/> | |
− | + | The third ethical issue is reducing a child to a numeric risk score can lead to an oversimplification of a complex social scenario. Predictive algorithms can input an enormous number of variables to make connections but cannot understand the human interactions that many child abuse victims will face.<ref name="silver"/> | |
− | + | ===Human Bias=== | |
+ | The human creation of algorithms raises ethical concerns of societal and [https://en.wikipedia.org/wiki/Cultural_bias cultural bias]. Although algorithms that feature machine learning interpret previous results and user input independently of direct manipulation by a programmer, humans continue to implement pre-programmed rules. Critics argue that algorithms, although unintentional, are intrinsically encumbered with the values of their creators. <ref>Luciano, F. (2016). The ethics of algorithms: Mapping the debate, big data & society. https://us.sagepub.com/en-us/nam/journal/big-data-society</ref> | ||
− | + | The primary ethical concern regarding the biases incorporated into algorithms is that they are difficult to find until a problematic result arises, which could be catastrophic for a child in danger. One study found that a child’s race was used in determining the likelihood of them being abused. These flawed parameters resulted in some automated decision-making software resulting in false positives up to 96% of the time.<ref>Quantzig. (2017). Predictive Analytics and Child Protective Services. https://www.quantzig.com/blog/predictive-analytics-child-protective-services</ref> | |
− | + | ==Ethical Standards== | |
+ | Given the stakes involved with child protection decisions, agencies utilizing these technologies have taken measures to make their processes ethically sound. | ||
− | + | Predictive models make decisions that can significantly impact a person’s life either positively or negatively, depending on the specific context and input. For this reason, there are widely accepted standards in place to determine the efficacy of a given algorithm at predicting [https://en.wikipedia.org/wiki/Child_abuse child endangerment].<ref name="PA"/> | |
− | + | ====Validity==== | |
+ | The validity of a predictive model is the measure of whether an algorithm is measuring what it is meant to measure.<ref name="PA"/> This is calculated as the number of true results ([https://en.wikipedia.org/wiki/False_positives_and_false_negatives#true_positive true positives] and true negatives) over the total samples analyzed, resulting in higher values for more accurate algorithms. | ||
− | + | ====Equity==== | |
+ | An important facet of any automated government decision-making is the equal treatment of cases across any demographic. In this regard, equity is the measure of variance in risk calculation across major geographic, racial, and ethnic groups to determine the applicability of an algorithm.<ref name="PA"/> | ||
− | + | ====Reliability==== | |
+ | Reliability is a measure of the consistency of results from different users of a predictive model when provided with the same information. This is important to social service agencies when attempting to maintain a policy among caseworkers and offices.<ref name="PA"/> | ||
− | + | ====Usefulness==== | |
+ | Usefulness is the measure of how applicable the results of an algorithm are to a case that is being investigated. Although this is not easily measured numerically, the following guidelines exist to help categorize an algorithm as useful: “When no potential exists for a particular predictive model to impact practice, improve systems or advance the well-being of children and families, then that model is inadequate.”<ref name="PA"/> | ||
− | + | ===Human Use=== | |
− | + | ||
− | + | ||
− | + | ||
− | + | ||
− | + | ||
− | + | ||
− | + | ||
− | + | ||
− | + | ||
− | = | + | |
− | + | ||
− | + | ||
− | + | ||
− | + | ||
− | + | ||
− | + | ||
− | + | ||
− | == Human | + | |
− | + | ||
− | + | ||
− | + | ||
− | + | ||
− | + | ||
− | + | ||
− | + | ||
− | + | ||
− | + | ||
− | + | ||
− | + | ||
− | + | ||
− | + | ||
− | + | ||
− | + | ||
− | + | ||
− | + | ||
− | + | ||
− | + | ||
− | + | ||
− | + | ||
− | + | ||
− | + | ||
− | + | ||
− | + | ||
− | + | ||
− | + | ||
− | + | ||
− | + | ||
− | + | ||
[[File:AutomationBias.png|200px|right|thumb|A graphic illustration of Automation Bias]] | [[File:AutomationBias.png|200px|right|thumb|A graphic illustration of Automation Bias]] | ||
− | Even the most powerful computer with a large quantity of data and a precise algorithm cannot be perfect, where a human | + | Even the most powerful computer with a large quantity of data and a precise algorithm cannot be perfect, where a human caseworker with experience must fill in the gaps. |
− | + | ||
− | + | ||
− | Predictive analysis can be an ally to health care professionals who use | + | An issue with these algorithms is [https://en.wikipedia.org/wiki/Automation_bias automation bias], the misconception that algorithms are neutral and inherently accurate. This phenomenon can result in caseworkers becoming over-reliant on automated decision-making software, overlooking any flaws present in the algorithmic process, and not making an effort to verify the results through other means.<ref name="coding"/> |
+ | Predictive analysis can be an ally to health care professionals who use numerical insight to inform their decision rather than entirely depend on it. The software is not capable of understanding the specific context nor the intricacies of human interaction to the point that it will always be 100% accurate.<ref name="PA"/><ref name="AC">Vaithianathan, R., & Putnam-Hornstein, E. (2017). Developing Predictive Models to Support Child Maltreatment Hotline ScreeningDecisions. <i>Allegheny County Methodology and Implementation</i>. Centre for Social Data Analytics, 48-56.</ref> | ||
− | + | =References= | |
− | + |
Latest revision as of 01:28, 11 April 2021
Automated decision-making regarding child protection refers to using software in an attempt to quantify the future possibility of harm for a child in a given context. This type of program is used along with caseworkers as a method of screening calls placed about possible domestic violence situations to determine the risk level of a child involved.[1] In the United States, this system has been deployed in Allegheny County, Pennsylvania, with some mixed results.[2] Child protection decision-making software is an application of predictive analytics, and some argue that it carries ethical concerns, some of which are unique to this specific application.[1]
Contents
Functionality
The system's goal is to apply various forms of trend identification to predict the likelihood of an event occurring in the future that could endanger a child.[3] New predictive tools take in vast amounts of administrative data and weigh each variable to identify situations that warrant intervention, and some are working to expose potential sources of bias and unfairness in these tools.[4]
Childhood protection algorithms vary from model to model, but often, as Stephanie Glaberson describes, these predictive analysis techniques use machine learning strategies to assess data and create the models. Glaberson describes this process as a product of the curated data and the programmers developing the machine learning algorithms. For example, it requires the developers to choose problem areas or factors for the predictive techniques to target and take into consideration.[3] These algorithms are critically described by some as "black box" algorithms, meaning the details and specifics are unclear, especially to those who are using the algorithm and were not involved in its creation.[3][4][5]
Examples
Pennsylvania
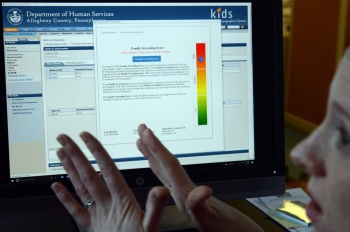
In August of 2016, Pennsylvania’s Allegheny County became the first in the United States to use automated decision-making software, the Allegheny Family Screening Tool (AFST), to supplement its existing child abuse hotline screening process. The tool offers a risk score as a “second opinion” to the call operator’s initial verdict, allowing them to change their mind over whether or not to flag the call as worthy of an investigation.[6] Flagging the call as worthy of investigation is referred to as "screening in," whereas offering outside resources and opting for no investigation is called "screening out."[7] This risk score provided by the AFST is based on two factors: re-referral (the likelihood that, upon a "screen out," the child or children will be referred back within two years) and placement (the likelihood that, upon a "screen in," the child or children will be removed from their home within two years).
A case study by Chouldechova et al. found the AFST to have implementation mistakes. Errors with the training and testing of the algorithm that created the models resulted in an overestimate of the model's performance results. In April of 2017, the research team began rebuilding the model to correct for these errors.[2] The AFST, along with other predictive analysis tools relating to child protection, has been criticized by some for the potential of bias or overcompensation.[6] However, supporters argue that it is not meant to replace human judgment, but to assist.[7]
Florida
In Florida, a non-profit organization called Eckerd Connects (formerly Eckerd Kids), in partnership with a for-profit company called Mindshare Technology, offers its own predictive analytics tool for child protection called Rapid Safety Feedback (RSF). This tool was more widely criticized than AFST due to high risk predictions and reportedly missing children that were high risk but not detected by RSF.[3][4] Further critiques came against Eckerd Connect and RSF after they refused to reveal details about their algorithm, even after the deaths of two children that had not been designated high risk by the RSF tool.[6] There are five states currently working with Eckerd Connects to adopt the RSF tool in their child welfare systems.[8]
United Kingdom
In the United Kingdom, some local councils have turned to algorithmic profiling to predict child abuse; these algorithms are designed to help take pressure off caseworkers and allow them to focus human resources elsewhere.[9] There are several reasons these councils have turned to technology in recent years. The first is pressure from 2014 media reports of failures within child protection systems.[4] The reports were based on a study that found that human caseworkers in the UK were committing three kinds of errors. Slow revision of initial judgments even in light of new evidence, confirmation bias, and witnesses bias (for example, doctors carried more weight than neighbors).[3] The councils believe that the algorithms may provide an unbiased opinion on which cases to investigate first. Additionally, budget cuts to welfare programs are the primary cause of departments turning to algorithms to assist a reduced number of available caseworkers. In some cases, there are no alternatives for high-quality child maltreatment centers.
These algorithms collect data on school attendance, housing association repairs, police records on antisocial behavior and domestic violence. Although many believe this data would help the algorithms, critics question using children's sensitive personal data without explicit permission. Data handling is a concern for many citizens in the UK as some believe the Data Protection Act is not equipped to handle data of this magnitude. Most councils are simply passing along alerting information to caseworkers, including school expulsion or domestic violence reports in a child's home. These risk alerts are intended to help caseworkers predict child abuse before it escalates. However, experts wonder if intervention in family life is beneficial in most situations and the risk of false positives impeding a family dynamic. [10]
The Sweetie Project
In 2013, the children’s rights organization Terre des Hommes launched a fake digital child called Sweetie, in an effort to use artificial intelligence to catch online child sex predators. This endeavor used a simulation of a child, completely rendered by computer graphics, named Sweetie. The current model of Sweetie 2.0 uses an automated chat function. It tracked, identified, and threatened users of the offense they were participating in. Sweetie 1.0 required investigators to participate in the chatroom dialogue in order to catch offenders. This allowed human eyes to effectively identify child abuse and take action. It also limited Sweetie’s ability to catch as many offenders as possible. With an automated chat function, Sweetie could track and catch significantly more offenders of virtual child abuse in sex tourism.[11] Despite initial reports that Sweetie would no longer be used, in 2019 the work was revived in a project known as #Sweetie 24/7.[12][13]
Controversy
The Sweetie Project has brought up ethical controversy about the legality of this practice of catching online pedophiles. Criminal laws on the degree of offense of online sex involving a child are unclear, especially when participants are from different countries. Professor Simone van der Hof also explores the question of whether the Sweetie technology constitutes entrapment. Since Sweetie is not yet an official tool for criminal prosecution, the validity of this automated technology for child abuse tracking is debatable.[11]
Morality Concerns
At least three major ethical concerns exist in applying predictive analytics to child protection.[14]. The first is a general concern regarding algorithms that perform machine learning, known as algorithmic transpaency. Algorithmic transparency refers to a program producing results that cannot be reverse-engineered due to a lack of information about the algorithm. Missing details about an algorithm's inner workings can result from proprietary software or the nature of machine learning.
Another concern with predictive learning in child protection is the prediction of rare events. Critical incidents where the abuse of a child could be fatal are near 2% of all events, which leads to an ethical dilemma of preferring false positives or negatives. An algorithm will never be perfect, which leads to the moral question of whether software should return false positives at the cost of resources or false negatives at the price of child safety.[14]
The third ethical issue is reducing a child to a numeric risk score can lead to an oversimplification of a complex social scenario. Predictive algorithms can input an enormous number of variables to make connections but cannot understand the human interactions that many child abuse victims will face.[14]
Human Bias
The human creation of algorithms raises ethical concerns of societal and cultural bias. Although algorithms that feature machine learning interpret previous results and user input independently of direct manipulation by a programmer, humans continue to implement pre-programmed rules. Critics argue that algorithms, although unintentional, are intrinsically encumbered with the values of their creators. [15]
The primary ethical concern regarding the biases incorporated into algorithms is that they are difficult to find until a problematic result arises, which could be catastrophic for a child in danger. One study found that a child’s race was used in determining the likelihood of them being abused. These flawed parameters resulted in some automated decision-making software resulting in false positives up to 96% of the time.[16]
Ethical Standards
Given the stakes involved with child protection decisions, agencies utilizing these technologies have taken measures to make their processes ethically sound.
Predictive models make decisions that can significantly impact a person’s life either positively or negatively, depending on the specific context and input. For this reason, there are widely accepted standards in place to determine the efficacy of a given algorithm at predicting child endangerment.[1]
Validity
The validity of a predictive model is the measure of whether an algorithm is measuring what it is meant to measure.[1] This is calculated as the number of true results (true positives and true negatives) over the total samples analyzed, resulting in higher values for more accurate algorithms.
Equity
An important facet of any automated government decision-making is the equal treatment of cases across any demographic. In this regard, equity is the measure of variance in risk calculation across major geographic, racial, and ethnic groups to determine the applicability of an algorithm.[1]
Reliability
Reliability is a measure of the consistency of results from different users of a predictive model when provided with the same information. This is important to social service agencies when attempting to maintain a policy among caseworkers and offices.[1]
Usefulness
Usefulness is the measure of how applicable the results of an algorithm are to a case that is being investigated. Although this is not easily measured numerically, the following guidelines exist to help categorize an algorithm as useful: “When no potential exists for a particular predictive model to impact practice, improve systems or advance the well-being of children and families, then that model is inadequate.”[1]
Human Use
Even the most powerful computer with a large quantity of data and a precise algorithm cannot be perfect, where a human caseworker with experience must fill in the gaps.
An issue with these algorithms is automation bias, the misconception that algorithms are neutral and inherently accurate. This phenomenon can result in caseworkers becoming over-reliant on automated decision-making software, overlooking any flaws present in the algorithmic process, and not making an effort to verify the results through other means.[3] Predictive analysis can be an ally to health care professionals who use numerical insight to inform their decision rather than entirely depend on it. The software is not capable of understanding the specific context nor the intricacies of human interaction to the point that it will always be 100% accurate.[1][17]
References
- ↑ 1.0 1.1 1.2 1.3 1.4 1.5 1.6 1.7 Russell, J. (2015). Predictive analytics and child protection: Constraints and opportunities. Child Abuse & Neglect, 46, 182-189. https://doi.org/10.1016/j.chiabu.2015.05.022
- ↑ 2.0 2.1 Chouldechova, A. (2018). A case study of algorithm-assisted decision making in child maltreatment hotline screening decisions. Conference on Fairness, Accountability, and Transparency, 81(1). Retrieved April 10, 2021, from http://proceedings.mlr.press/v81/chouldechova18a/chouldechova18a.pdf
- ↑ 3.0 3.1 3.2 3.3 3.4 3.5 Glaberson, S. K. (2019). Coding Over the Cracks: Predictive Analytics and Child Protection. Fordham Urban Law Journal, 46(2), 306-363. Retrieved April 2, 2021, from https://ir.lawnet.fordham.edu/ulj/vol46/iss2/3
- ↑ 4.0 4.1 4.2 4.3 Keddell, E. (2019). Algorithmic Justice in Child Protection: Statistical Fairness, Social Justice and the Implications for Practice. Social Sciences, 8(10), 281. https://doi.org/10.3390/socsci8100281
- ↑ Merriam-Webster. (n.d.). Black Box. In Merriam-Webster.com dictionary. Retrieved April 10, 2021, from https://www.merriam-webster.com/dictionary/black%20box
- ↑ 6.0 6.1 6.2 Hurley, D. (2018, January 2). Can An Algorithm Tell When Kids Are in Danger? The New York Times. https://www.nytimes.com/2018/01/02/magazine/can-an-algorithm-tell-when-kids-are-in-danger.html
- ↑ 7.0 7.1 Allegheny County. (n.d.). The Allegheny Family Screening Tool. https://www.alleghenycounty.us/Human-Services/News-Events/Accomplishments/Allegheny-Family-Screening-Tool.aspx
- ↑ Eckerd Connects. Eckerd Rapid Safety Feedback. Retrieved April 11, 2021, from https://eckerd.org/family-children-services/ersf/
- ↑ McIntyre, N., & Pegg, D. (2018, September 16). Councils use 377,000 people's data in efforts to predict child abuse. Retrieved April 2, 2021, from https://www.theguardian.com/society/2018/sep/16/councils-use-377000-peoples-data-in-efforts-to-predict-child-abuse
- ↑ Brown, P., Gilbert, R., Pearson, R., Feder, G., Fletcher, C., Stein, M., Shaw, T., Simmonds, J. (2018, September 19). Don't trust algorithms to predict child-abuse risk | letters. Retrieved April 2, 2021, from https://www.theguardian.com/technology/2018/sep/19/dont-trust-algorithms-to-predict-child-abuse-risk
- ↑ 11.0 11.1 van der Hof, S., Georgieva, I., Schermer, B., Koops, B.-J. (Eds.). (2019). Sweetie 2.0: Using artificial intelligence to fight webcam child sex tourism [Abstract]. Asser Press. https://www.springer.com/gp/book/9789462652873
- ↑ Crawford, A. (2013, November 5). Computer-generated 'Sweetie' catches online predators. BBC News. https://www.bbc.com/news/uk-24818769
- ↑ Terre des hommes. (n.d.). Sweetie, our weapon against child webcam sex. https://www.terredeshommes.nl/en/programs/sweetie
- ↑ 14.0 14.1 14.2 Church, C.E. & Fairchild, A.J. (2017). In Search of a Silver Bullet: Child Welfare's Embrace of Predictive Analytics. Juvenile & Family Court Journal, 68(1), 67-81. https://doi-org.proxy.lib.umich.edu/10.1111/jfcj.12086
- ↑ Luciano, F. (2016). The ethics of algorithms: Mapping the debate, big data & society. https://us.sagepub.com/en-us/nam/journal/big-data-society
- ↑ Quantzig. (2017). Predictive Analytics and Child Protective Services. https://www.quantzig.com/blog/predictive-analytics-child-protective-services
- ↑ Vaithianathan, R., & Putnam-Hornstein, E. (2017). Developing Predictive Models to Support Child Maltreatment Hotline ScreeningDecisions. Allegheny County Methodology and Implementation. Centre for Social Data Analytics, 48-56.