Artificial Intelligence in Hiring and Recruitment
Artificial Intelligence (AI) in Hiring and Recruitment is an emerging field that involves the use of AI-enabled software and algorithms to assist in the recruitment and hiring process. The technology can be used in a number of ways, including sourcing candidates, assessing resumes and applications, conducting initial interviews, and providing data-driven insights for HR professionals. Proponents of AI in HR argue that it can streamline the hiring process, reduce bias, and improve the quality of hires. However, opponents express concerns about the potential for AI to reinforce existing biases, reduce the role of human decision-making, and perpetuate discrimination.
Research has shown that AI-enabled hiring software can increase the efficiency of the hiring process and provide access to broader and more diverse pools of candidates. However, there are concerns about the accuracy of the data being used and the level of control over the algorithmic candidate matching process. The implementation of AI in HR can vary depending on the industry and the specific hiring scenario, and may redefine the role of HR professionals as some aspects of the hiring process become automated or augmented by AI[1].
Despite challenges, AI in hiring continues to gain popularity. AI-powered recruitment tools are being continuously developed to reduce bias, increase efficiency, and improve the quality of hires[2].
Contents
Common use cases of AI in hiring and recruitment
Resume screening
Resume screening algorithms have been developed to automate the process of sorting resumes based on specific skill sets required by companies. The traditional method of manual sorting is time-consuming, and there is a higher probability of errors due to human intervention. AI has been proposed as a solution to this problem– systems are trained to recognize keywords or special skills required by the company, and resumes are scanned and sorted accordingly. The AI-based system shortlists resumes that match the company's requirements and forwards them to the respective HR for further recruitment processes. The system segregates resumes as selected or rejected and stores them in the form of excel sheets, forming different folders that store data regarding selected and rejected resumes. This process is highly efficient compared to manual sorting, as it reduces errors and saves time.[3].
Chatbots
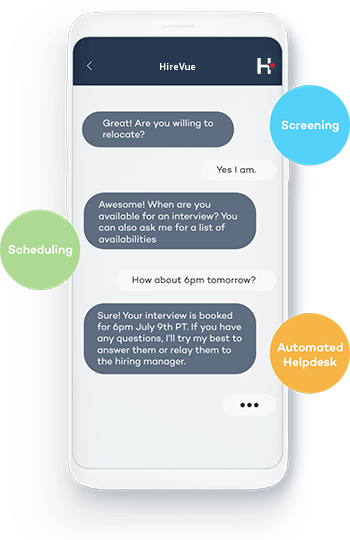
Chatbots are becoming increasingly popular in the field of AI for hiring, as they also eliminate routine work in the recruitment process. Chatbots simplify the first stage of screening applications, interpreting resumes, answering usual inquiries, and gathering a wide range of data for human selection representatives to analyze. Chatbots can also automate end-to-end recruitment processes, attendance tracking, goal tracking, performance reviews, employee surveys, balance leaves, and other activities, enabling HR managers to succeed in the digitalized era. Additionally, they can facilitate sequence questions for the applicant to answer, adopt smart methods for collecting data of the candidate, and provide new insights for better candidate profiles. Automated Facebook recruitment chatbots, for example, prompt potential applicants to opt-in for the company's career page and submit their application, simplifying the recruitment process.[5].
Personality and cognitive assessments
Personality testing has been an essential part of industrial-organizational and vocational psychology for over 85 years. AI-enabled personality assessment has become increasingly prevalent in hiring processes, with the aim of predicting whether an employee will engage in undesirable job behaviors and whether they are trustworthy. Several assessments have been developed, including Cattell's 16-PF, the California Psychological Inventory (CPI), and the NEO Personality Inventory (NEO PI), which assess personality traits important for social interaction, personality dimensions based on the "Big Five," or Five Factor Model, including openness, agreeableness, neuroticism, extraversion, and conscientiousness[6].
With usage of convolutional neural network models, AI can successfully recognize human nonverbal cues and attribute personality traits based on features extracted from video interviews. An end-to-end AI interviewing system was developed in this study using asynchronous video interview (AVI) processing and a TensorFlow AI engine to perform automatic personality recognition (APR). The study involved 120 real job applicants, and the AI-based interview agent achieved an accuracy between 90.9% and 97.4% in recognizing the “big five” personality traits of an interviewee. The use of AI in personality assessment can supplement or replace existing self-reported personality assessment methods used by employers, which can be distorted by job applicants to achieve socially desirable effects[7].
Personalization
AI-enabled applications are also deployed in other areas of the employment life cycle, such as socialization, training, coaching, development, performance management, reward management, and tracking an employee's progress until off-boarding. The unitary approach to managing the entire workforce has transformed into a more integrated and personalized approach, taking HR analytics into account for decision-making on all aspects of an employee's employment life cycle. AI-enabled calibrated coaching allows an employee to complete a short online assessment and schedule a set of learning recommendations to adjust for required behaviors against their current skill levels[8].
Effects of AI in hiring and recruitment
Efficiency
The use of AI in HR functions promotes cost-effectiveness and efficiencies, allowing HR managers and planners to make the best selections or decisions from various alternatives with reduced bias. AI-enabled HR applications aid decision-making through data mining, pattern recognition, aggregations, and clustering based on context efficiently, allowing HR practitioners to manage a diverse geographically dispersed workforce and track their critical movements and utilization rates within their employment life cycle[9].
The use of AI in hiring can increase efficiency, but there are also potential risks. For example, decisions could be made too quickly without enough reflection on the consequences that might follow. Researchers have studied problems of complacency and automation bias within the field of human factors. Additionally, AI-enabled sourcing software could disempower recruiters if the software's increasing ease of use via algorithmically driven suggestions limits the level of control the tools can provide[10].
Bias
AI-powered recruiting tools have the potential to mitigate human bias and help make the hiring process fairer. However, there are concerns over the use of machine learning in hiring software that may perpetuate or even exacerbate existing biases.
To address these concerns, several startups have emerged that claim to have designed and trained their AI models to specifically address various sources of systemic bias in the recruitment pipeline. The strategies adopted by these companies include scrubbing identifying information from applications, relying on anonymous interviews and skill-set tests, and even tuning the wording of job postings to attract as diverse a field of candidates as possible. For instance, GapJumpers offers a platform for applicants to take “blind auditions” designed to assess job-related skills. The startup uses machine learning to score and rank each candidate without including any personally identifiable information. This method helps reduce reliance on resumes, which, as sources of training data, are “riddled with bias.”
However, paying close attention to the design and training of the system has been criticized as insufficient, with claims that AI software will almost always require constant human oversight[11].
can also have discriminatory effects on legally protected groups such as women, ethnic minorities, and people with disabilities. The issue of algorithmic bias has become a significant concern, as evidenced by mounting evidence of its adverse effects. For instance, facial recognition technologies have been shown to assign more negative emotions to black men’s faces than to white men’s faces. Moreover, speech interpretation algorithms have learned to associate female names with domestic duties. It is worth noting that bias in machine learning algorithms is often because they learn from biased human decision-makers. Therefore, algorithmic bias can be easier to reduce than human bias if it is adequately regulated[12].
It is important to note that even if an algorithm is biased, it may be an improvement over the current status quo. For instance, a 2018 study explored the possibility of using an algorithm trained on historical criminal data to predict the likelihood of criminals re-offending to make bail decisions. The authors were able to reduce crime rates by 25% while reducing instances of discrimination in jailed inmates. However, the gains highlighted in this research would only occur if the algorithm was actually making every decision, which is unlikely to happen in the real world as judges would likely prefer to choose whether or not to follow the algorithm’s recommendations[13].
However, bias can also appear for unrelated reasons. For example, a recent study showed that an algorithm delivered ads promoting STEM jobs more to men than to women not because men were more likely to click on them, but because women are more expensive to advertise to. Since companies price ads targeting women at a higher rate (women drive 70% to 80% of all consumer purchases), the algorithm chose to deliver ads more to men than to women because it was designed to optimize ad delivery while keeping costs low[14].
To mitigate the potential for bias, standards for model explainability and transparency have been proposed, including the use of data sheets that document all aspects of the model's provenance. Additionally, stakeholder consultation and engagement are important components of ethical AI development, as those affected by AI should be able to participate in decisions over its development[15].
Legal implications
While voluntary standards for ethical AI are being developed, statutory regulation may be necessary to provide necessary safeguards. In the United States, civil rights legislation provides some protection for workers against discriminatory AI in hiring, promotions, and pay decision making, but does little to constrain AI being used to increase employer dominion over workers through new tools for monitoring and controlling worker activity. In Europe, the General Data Protection Regulation (GDPR) provides some protections for workers, but the absence of enforcement mechanisms has been criticized.
As AI hiring models raise a set of legal challenges, including issues around legal accountability for AI decisions, new laws may be necessary[16].
Public bodies in the EU and the UK are increasingly paying attention to AI hiring. However, the regulation of AI in Europe is still at an embryonic stage. Many algorithmic tools sold on the European market are developed in the United States. As a result, algorithm vendors’ bias mitigation efforts are generally informed by US law, which differs substantially from European equality law. For instance, US-based algorithm vendors often advertise their compliance with the ‘four-fifths’ rule, which requires employers to justify the use of apparently neutral hiring practices if these practices result in a selection rate for one group which is less than four-fifths that of another group. However, European equality law has expressly eschewed the use of strict numerical thresholds, and an algorithm that complies with the four-fifths rule could violate European equality law. Moreover, the absence of a federal data protection regime in the United States means that little consideration has been given to the dual application of data protection law and equality law in this space. The opacity of automated hiring continues to pose a significant challenge to enforcement[17].
Integration
AI can also be integrated with other technologies such as IoT, big data, and blockchain technologies to enhance HR functions. For instance, using big data analytics can help HR practitioners to make more informed decisions based on data from multiple sources. The use of blockchain technology can ensure that the data is secured and tamper-proof, thereby increasing transparency and trust in the HR processes.
The integration of AI with other technologies can also help firms design impactful AI applications and platforms that enhance their employees' work commitment, job satisfaction, and overall employee experience (EX) using novel EX platforms as part of their digitalized HR ecosystem. EX is a key antecedent for employee engagement, and the use of diverse digitized AI-enabled and other technology platforms can create diverse touchpoints as part of an employee's life cycle to deliver high levels of EX. The technologies promote HR functions' cost-effectiveness and efficiencies while allowing HR managers and planners to exercise their human cognition and judgment to make the best selections or decisions from various alternatives with reduced bias[18].
Notable examples of use
Amazon
In an effort to streamline its recruitment process and find the best candidates, Amazon created an experimental AI recruiting tool that combed the web for potential candidates, rating them on a scale of one to five stars. However, the algorithm learned to systematically downgrade women’s CVs for technical jobs, such as software developer, revealing an inherent gender bias in the AI model.
Because the AI algorithm used all CVs submitted to the company over a ten-year period to learn how to spot the best candidates, it quickly spotted male dominance and thought it was a factor in success, creating a pattern of sexism against female candidates. Amazon eventually shut down the AI tool after being unable to find a way to make it gender-neutral[19].
Future iterations
In response to use of (AI) in hiring has raising concerns about the potential for bias in decision-making, a hybrid setting model has been discussed. A hybrid setting involves a human decision-maker who can choose whether to utilize a trained model's inferences to help select candidates from written biographies. A large-scale user study was conducted leveraging a recreated dataset of real bios from prior work, where humans predicted the ground truth occupation of given candidates with and without the help of three different NLP classifiers. The results demonstrated that while high-performance models significantly improve human performance in a hybrid setting, some models mitigate hybrid bias while others accentuate it. Both performance and bias are important for real-world deployment and exhibit different social implications in practice. The interaction between human and machine is crucial in hybrid performance, requiring an evaluation of a different nature that looks at how humans choose to conform to specific models. While it has been investigated how model accuracy transfers to hybrid accuracy, the way predictive bias inherent in ML models transfer to human decisions is not well understood. Specifically, it is not clear how biases from different model architectures would influence human bias or whether a more biased model would ultimately propagate to a human decision-maker at a higher rate than a less biased one[20].
References
- ↑ Li, Lan, et al. "Algorithmic hiring in practice: Recruiter and HR Professional's perspectives on AI use in hiring." Proceedings of the 2021 AAAI/ACM Conference on AI, Ethics, and Society. 2021
- ↑ Ahmed, Owais. "Artificial intelligence in HR." International Journal of Research and Analytical Reviews 5.4 (2018): 971-978.
- ↑ Dixit, V. V., et al. "Résumé sorting using artificial intelligence" International Journal of Research in Engineering, Science and Management 2.4 (2019): 423-425.
- ↑ 'Hirevue Essential Guide to Chatbots in Recruitment.'. Hirevue. Retrieved Feb 20, 2023, from https://www.hirevue.com/blog/hiring/recruitment-chatbot
- ↑ Nawaz, Nishad, and Anjali Mary Gomes. "Artificial intelligence chatbots are new recruiters" IJACSA) International Journal of Advanced Computer Science and Applications 10.9 (2019).
- ↑ Gordon, Nicolette, and Kimberly Weston Moore. "The Effects of Artificial Intelligence (AI) Enabled Personality Assessments During Team Formation on Team Cohesion." Information Systems and Neuroscience: NeuroIS Retreat 2022 (2022): 311-318.
- ↑ Suen, Hung-Yue, Kuo-En Hung, and Chien-Liang Lin. "TensorFlow-based automatic personality recognition used in asynchronous video interviews." IEEE Access 7 (2019): 61018-61023.
- ↑ Malik, Ashish, Praveena Thevisuthan, and Thedushika De Sliva. "Artificial Intelligence, Employee Engagement, Experience, and HRM." Strategic human resource management and employment relations: An international perspective. Cham: Springer International Publishing, 2022. 171-184.
- ↑ Malik, Ashish, Praveena Thevisuthan, and Thedushika De Sliva. "Artificial Intelligence, Employee Engagement, Experience, and HRM." Strategic human resource management and employment relations: An international perspective. Cham: Springer International Publishing, 2022. 171-184.
- ↑ Li, Lan, et al. "Algorithmic hiring in practice: Recruiter and HR Professional's perspectives on AI use in hiring." Proceedings of the 2021 AAAI/ACM Conference on AI, Ethics, and Society. 2021
- ↑ Hsu, Jeremy. "Can AI hiring systems be made antiracist? Makers and users of AI-assisted recruiting software reexamine the tools' development and how they're used-[News]." IEEE Spectrum 57.9 (2020): 9-11.
- ↑ Kelly-Lyth, Aislinn. "Challenging biased hiring algorithms." Oxford Journal of Legal Studies 41.4 (2021): 899-928.
- ↑ Lavanchy, Maude. "Amazon’s sexist hiring algorithm could still be better than a human." The Conversation (2018).
- ↑ Lavanchy, Maude. "Amazon’s sexist hiring algorithm could still be better than a human." The Conversation (2018).
- ↑ Charlwood, Andy, and Nigel Guenole. "Can HR adapt to the paradoxes of artificial intelligence?." Human Resource Management Journal 32.4 (2022): 729-742.
- ↑ Charlwood, Andy, and Nigel Guenole. "Can HR adapt to the paradoxes of artificial intelligence?." Human Resource Management Journal 32.4 (2022): 729-742.
- ↑ Kelly-Lyth, Aislinn. "Challenging biased hiring algorithms." Oxford Journal of Legal Studies 41.4 (2021): 899-928.
- ↑ Malik, Ashish, Praveena Thevisuthan, and Thedushika De Sliva. "Artificial Intelligence, Employee Engagement, Experience, and HRM." Strategic human resource management and employment relations: An international perspective. Cham: Springer International Publishing, 2022. 171-184.
- ↑ Lavanchy, Maude. "Amazon’s sexist hiring algorithm could still be better than a human." The Conversation (2018).
- ↑ Peng, Andi, et al. "Investigations of performance and bias in human-AI teamwork in hiring." Proceedings of the AAAI Conference on Artificial Intelligence. Vol. 36. No. 11. 2022.